Big data analytics services
-
Get the full picture, fast. Combine massive datasets from across your business — real-time, historical, and external — for a single, accurate view.
-
Use advanced AI to crunch petabytes of data points, revealing patterns humans can’t see to predict market shifts and customer needs with uncanny accuracy.
-
Tap into cutting-edge tech without the headaches. Deploy powerful cloud big data analytics platform in days, not months.
-
Handle an explosion of data from all sources — web, mobile, IoT, and more. Serve insights to thousands of users without breaking a sweat.
Value we deliver to our clients
-
50+
delivered big data solutions
-
15+
dedicated big data experts at your service
-
20%
faster time to market with AI-powered software development
Yalantis big data analytics services
-
Sales and marketing analytics
Sales and marketing analytics
Sales and marketing analytics to predict trends across customer interactions
- Predict customer behavior with advanced ML models
- Set the right prices for thousands of SKUs in seconds
- Automatically personalize marketing for each customer
- Analyze multi-channel customer interactions to map complex buyer journeys
- Forecast sales trends with historical data and external factors
- Identify new market opportunities from competitor data analysis
-
Financial risk analytics
Financial risk analytics
Financial risk analytics to protect assets using large-scale data
- Detect fraud in real time across millions of transactions
- Stress-test your portfolio against hundreds of what-ifs
- Assess credit risk by analyzing diverse data sources
- Ensure compliance by automatically monitoring evolving global regulations
- Predict operational risks by analyzing patterns in big data
- Optimize investment portfolios using AI-driven market data analysis
-
Customer analytics
Customer analytics
Customer analytics for real-time personalizations
- Segment customers based on petabytes of behavioral data
- Predict long-term customer value using advanced analytics
- Personalize product recommendations in real time for millions of users
- Analyze customer feedback from social media, surveys, call logs, and chatbots simultaneously
- Improve customer retention with predictive churn models
-
Operational analytics
Operational analytics
Operational analytics to optimize complex, global supply chains
- Predict equipment failures using IoT sensor data and machine learning
- Improve delivery routes with large-scale location data
- Forecast demand by processing diverse, high-volume data sources
- Enhance quality control via quick production data analysis
- Reduce energy consumption across complex industrial systems
-
HR analytics
HR analytics
HR analytics to forecast workforce needs
- Identify employees that are likely to leave before they do
- Hire top candidates with automatic CV screening and predict candidate success
- Plan workforce needs with predictive analytics and external labor market data
- Measure and improve employee engagement through continuous feedback analysis
- Personalize learning and development programs based on individual and aggregate data
Discover the right analytics solution for your business
Case studies from our big data analytics company
Data Modernisation:
Unlock your business data’s potential with a modern, cost-effective system that makes data accessible, actionable, and easy for your team to embrace.
Insights into big data and analytics solutions
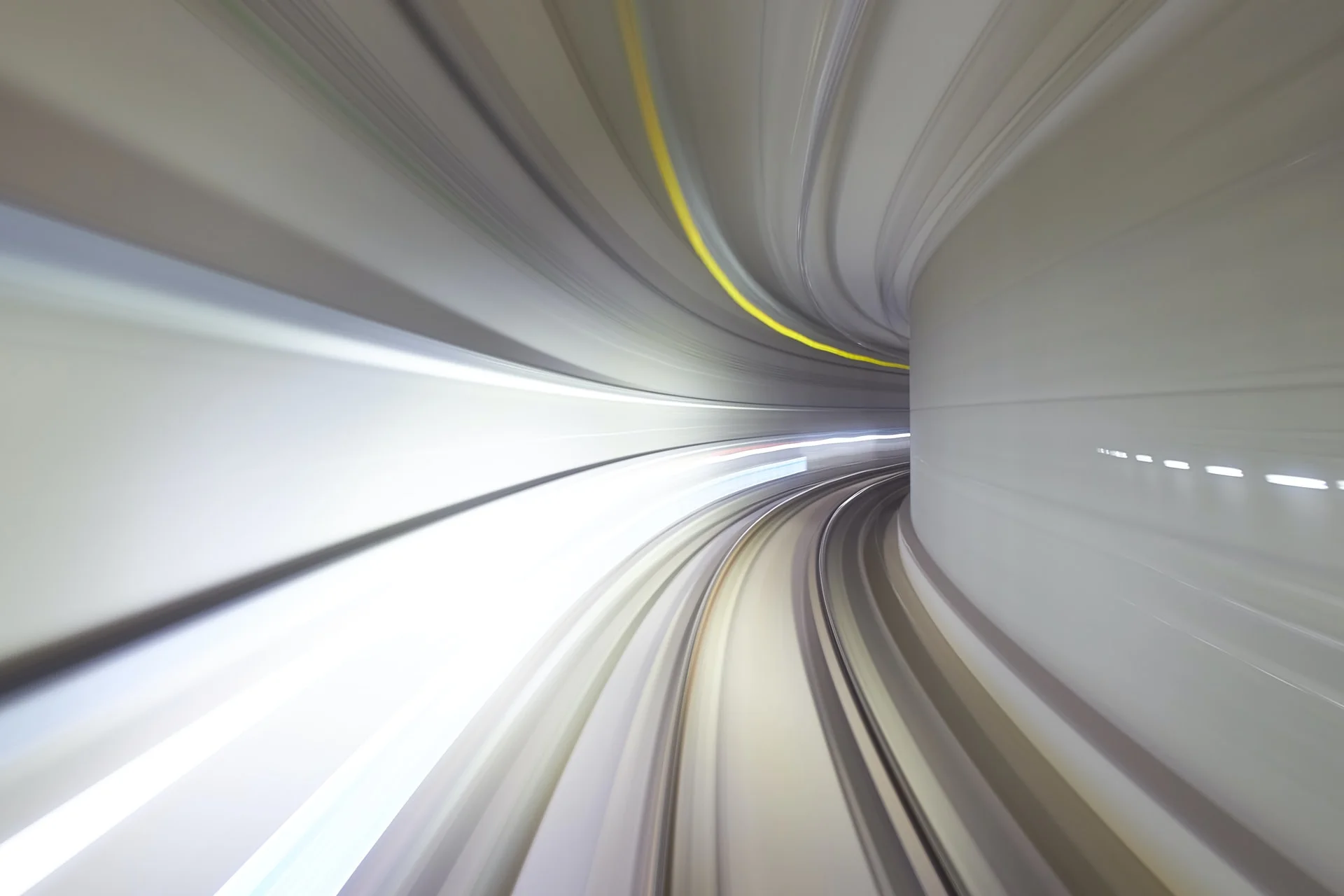
How to ensure efficient real-time big data analytics
Find out key stages of handling real-time big data efficiently, learn what tools and technologies to use to streamline this process.
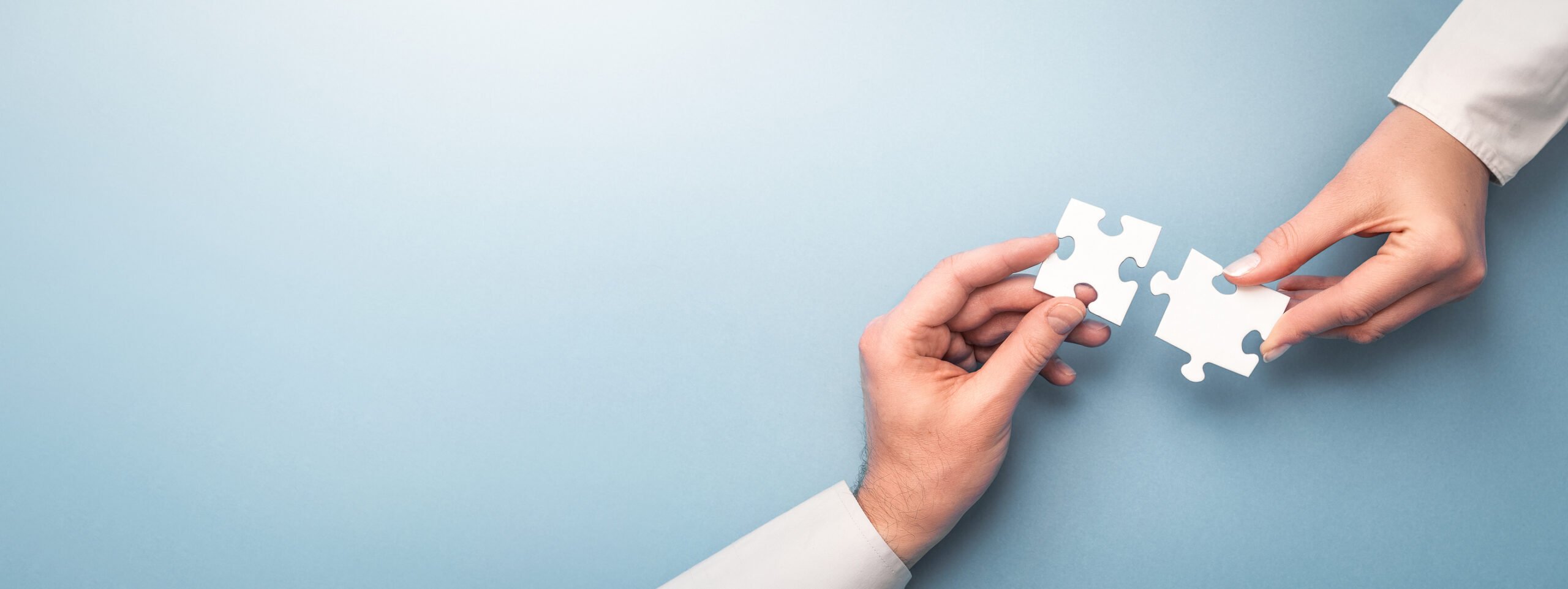
An enterprise data governance framework: Solve your biggest challenges with data security, quality, compliance
Is data chaos costing your business growth and innovation? Discover how to tackle data challenges around security, consistency, quality, and compliance with a single solution.
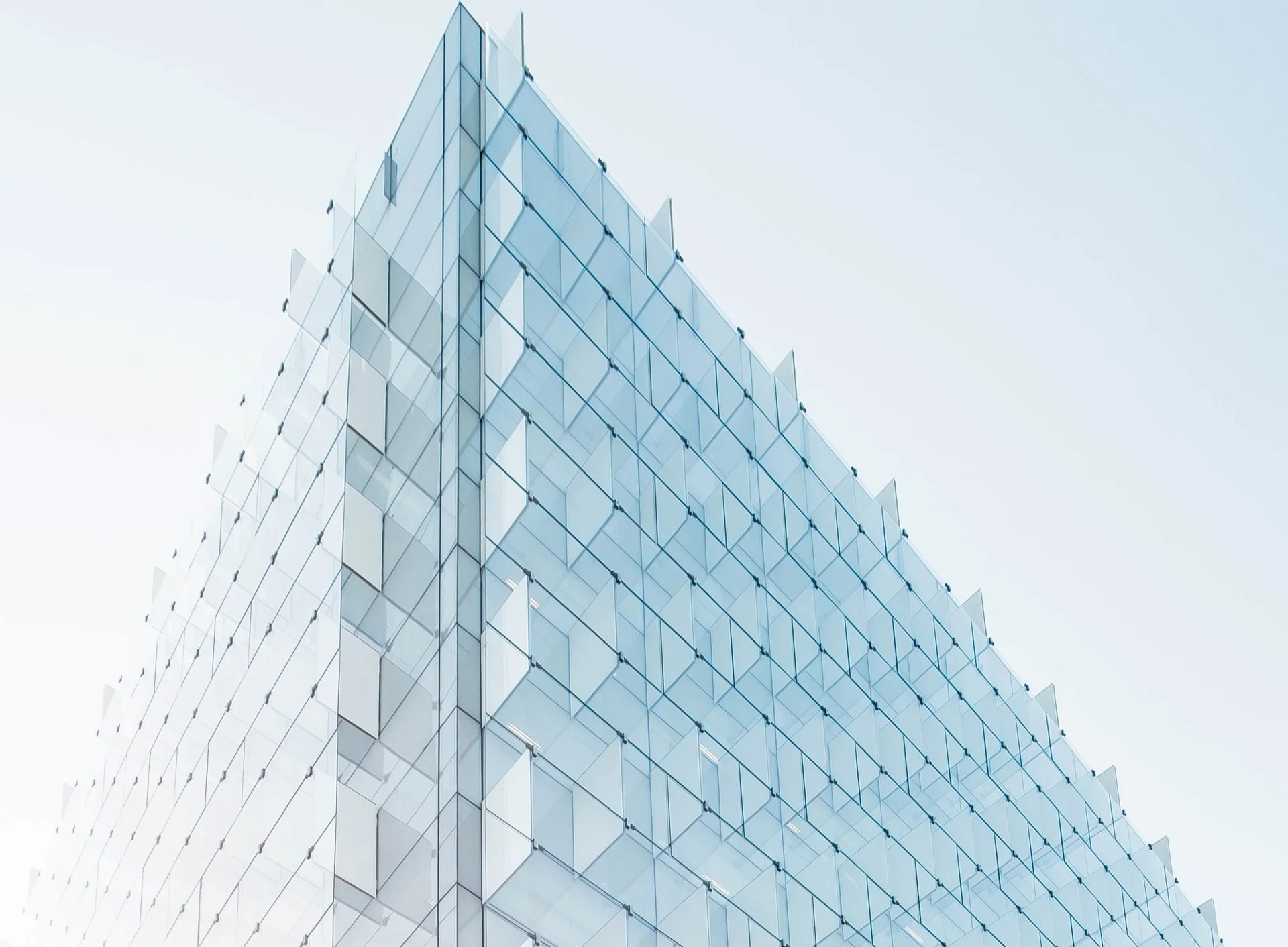
Central repository for your data: data mart, data warehouse, or data lake?
Learn the differences between a data warehouse, a data mart, and a data lake and pick the right data repository to cover your industry-specific needs.
How can you ensure that our big data analytics efforts are aligned with business goals?
Aligning data analytics with your business goals is at the core of our approach. Here’s how our team ensures this alignment:
- Goal assessment: We understand your business objectives and KPIs.
- Data audit: We evaluate your existing data sources and identify any gaps.
- Strategy development: We create a tailored analytics strategy that directly supports your business goals.
- KPI mapping: We link each analytics initiative to specific business outcomes.
- Regular reviews: We conduct periodic assessments to ensure ongoing alignment.
- Executive reporting: We provide clear, goal-oriented reports to demonstrate the impact of big data analytics on your business objectives.
What is the expected ROI from implementing advanced analytics?
Based on our experience, clients can expect significant returns in three months, including noticeable improvements in operational efficiency and customer satisfaction. For example, our 3PL client achieved better supply chain decision-making and reduced operational costs in this time frame through:
- streamlining data processes with real-time data aggregation, effective unstructured data analysis, and improved information accessibility
- improving decision-making by converting raw data from various sources into valuable insights
- increasing customer satisfaction by improving service quality and visibility
We’ll define clear objectives and track ROI throughout our engagement. The value of our partnership extends beyond financial returns and can include better decision-making by predicting outcomes along with an improved customer experience.
How will you ensure the security and privacy of our data during analytics processes?
We protect your data by:
- encrypting it at rest and in transit
- having an incident response plan in place
- anonymizing personal data when applicable
- performing regular security audits and penetration testing
- helping you establish robust data governance policies and procedures
- using secure, industry-leading cloud platforms with built-in security features
- ensuring all processes comply with relevant laws and regulations (GDPR, CCPA)
- implementing strict role-based access controls and multi-factor authentication
What if we already have a data analytics team?
Our role is to complement and enhance your existing capabilities. Here’s how we can work together:
- Skills gap analysis: Identifying areas where we can provide advanced expertise to supplement your team’s skills
- Knowledge transfer: Working alongside your team, sharing our expertise and best practices
- Advanced techniques: Introducing cutting-edge analytics methods like machine learning and AI, if not already in use
- Scaling and performance: Helping to optimize your analytics processes for scaling big data processes
- Tool integration: Helping you integrate advanced analytics tools with your existing technology stack
- Specialized projects: Taking on specific high-complexity projects while your team focuses on day-to-day operations
- Strategic guidance: Providing high-level strategic advice to help your team more closely align with business objectives
What tools and technologies do you use for big data analytics?
We work with a wide range of big data analytics tools and technologies, including:
- Apache Spark for large-scale data processing and machine learning
- Hadoop ecosystem (HDFS, Hive, HBase) for distributed storage and processing
- Python for statistical analysis and data science
- Tableau and Power BI for data visualization
- Kafka and Flink for real-time data streaming analytics
- Elasticsearch for fast, scalable search and analytics
- Snowflake and Google BigQuery for a cloud-based data warehouse
Note that we’re not limited to these technologies in our big data analytics services and can work with or recommend other tools based on your specific big data infrastructure needs.
Do you offer ongoing support and training for our team?
Absolutely. We believe in empowering your team to get the most out of your big data analytics investment. Our support and training include:
- Hands-on workshops: Practical training sessions on tools and methodologies
- Custom documentation: Detailed guides tailored to your specific analytics setup
- Knowledge transfer sessions: Ensuring your team understands the why behind our analytics approaches
- Regular check-ins: Periodic meetings to address any questions or challenges
- Skill development plans: Helping you identify areas for team growth and providing resources for continual learning
- Updates and upgrades: Keeping you informed about relevant new technologies and helping you integrate them when beneficial
Why businesses turn to big data analytics service providers: Scaling capabilities and AI/ML integration
To make the most of their data sets (that grow in volume, complexity, and velocity), businesses need to focus on two key things: making their big data systems bigger and smarter. That’s where scaling analytics and bringing in artificial intelligence (AI) and machine learning (ML) come into play. Many companies embrace big data services and turn to big data analytics service providers to navigate these challenges using their expertise and specialized tools.
Scaling analytics capabilities: Growing your big data muscle
Scalability is about making sure your systems can handle more data and more users as your business grows. It’s like designing an office space that can easily accommodate additional workstations and employees, rather than being locked into a fixed layout that becomes overcrowded as the company expands.
Scalability is crucial for businesses of all sizes, but especially for those experiencing rapid growth or dealing with large amounts of data. Think e-commerce platforms handling millions of transactions, social media networks processing billions of posts, or healthcare systems managing patient data across multiple hospitals.
Here’s how big data analytics service providers help enterprises tackle the scalability challenge:
- Embracing the cloud: Using cloud platforms like AWS, Azure, and Google Cloud that act as giant, flexible data centers and grow with your business needs. With cloud platforms, you can easily increase or decrease computing power, storage, and other resources on demand without investing in physical hardware. For example, during peak periods, you can quickly scale up processing power, then scale it down when demand lessens.
- Implementing scalable NoSQL databases: Guiding companies in choosing and setting up databases like MongoDB or Cassandra that are designed to handle large amounts of diverse data across many devices.
- Deploying smart data processing tools: Implementing technologies like Apache Spark and Apache Flink. These frameworks are key for organizations dealing with data at scale, allowing for both batch and real-time data processing.
- Architecting flexible systems: Designing microservices and event-driven architectures, creating interconnected services that can scale independently as needed.
For example, Yalantis helped a large US shipping company set up a scalable system to convert raw data from multiple sources (customer logs, supply chain systems, RFID tags, and IoT devices) into valuable information to support decision-making. We used:
- Amazon S3 to provide unlimited and low-cost scalability for the client’s serverless data lake
- Amazon QuickSight to provide a scalable and serverless business intelligence (BI) service for data visualization
Integrating AI and machine learning: Making your data smarter
Once a robust big data system is in place to handle vast amounts of information and grow easily, the next step could be using AI and machine learning to extract meaningful insights. Big data analytics service providers play a crucial role in this integration. Let’s explore how, using Yalantis’ work with a major US logistics company as an example. Our client struggled with effectively analyzing large amounts of unstructured data.
For this client, Yalantis integrated several key big data analytics components using Amazon Web Services (AWS):
- A data lake for centralized data storage (AWS Lake Formation)
- Real-time data extraction for up-to-the-minute information (AWS Data Exchange, Kinesis Data Firehose)
- Data visualization for an intuitive understanding of supply chain operations, customer behavior, and operational efficiency (AWS QuickSight)
The cherry on top was embedding functionality to forecast business trends. We used Amazon SageMaker for machine learning and predictive analytics on large-scale data.
Now, let’s see some real-life examples of how businesses put AI and ML to work:
- Automated insight generation: Machine learning models can quickly identify patterns and anomalies and analyze data in complex datasets. For example, Netflix uses ML algorithms to analyze viewing history and provide personalized content recommendations, increasing customer retention and generating $1 billion in value annually.
- Predictive analytics: By combining historical data with ML models, businesses can forecast trends and make data-driven decisions. Scotiabank, a Canadian digital bank, uses big data analytics to generate customer offers in hours instead of weeks, allowing them to respond quickly to changing customer needs.
- Real-time decision-making: The combination of streaming data processing and machine learning enables instant analytics and decision-making. This is crucial in areas like fraud detection, recommendation systems, and IoT applications. For instance, Command Alkon, a construction logistics company, implemented a real-time data analytics platform to manage material deliveries efficiently, providing users with critical insights in seconds.
- Natural language processing (NLP): NLP techniques analyze unstructured text data from sources like customer feedback and social media, opening up new avenues for insights.
- AutoML and model management: As ML use grows, tools for automating model development and managing models in production are becoming essential for scaling AI-driven analytics across organizations.
Note, however, that successful AI/ML integration requires not just technical implementation but also organizational changes to foster a data-driven culture and ensure ethical use of AI.
Challenges in scaling big data analytics and integrating AI/ML
While scaling analytics capabilities and integrating AI/ML offer great benefits, they come with their own set of challenges:
- Data quality and governance: As analytics capabilities scale, ensuring data quality and maintaining robust governance becomes complex but also increasingly critical. Poor data quality can lead to inaccurate business insights and decisions, while weak data governance can result in data breaches or non-compliance with regulations.
- Skills gaps: There’s a growing demand for professionals who understand both domain-specific analytics and the latest innovations in AI/ML technologies. This shortage can slow down implementation of advanced analytics solutions and limit an organization’s ability to fully use its data.
- Ethical considerations: As AI becomes more prevalent in analytics, organizations must address issues of bias, fairness, and transparency in their models and decisions. Failing to do so can lead to discriminatory outcomes, reputational damage, and loss of customer trust.
- Integration with existing systems: Many organizations struggle with integrating big data technologies and new, scalable analytics capabilities with legacy systems and processes. This can result in data silos, inefficiencies, and difficulty achieving a unified view of the business.
These challenges can be daunting, especially for organizations that lack in-house expertise in big data analytics and AI/ML. This is where the concept of big data analytics as a service comes into play, offering a solution that addresses many of these hurdles.
What is big data analytics as a service?
Big data analytics as a service (BDAaaS) is a cloud-based service model that provides end-to-end big data analytics solutions. This includes collecting and processing large datasets, performing advanced analytics, and generating actionable insights from both structured and unstructured data.
BDAaaS offers businesses access to sophisticated analytics tools, scalable computing infrastructure, and data science expertise without the complexities and costs of building and maintaining on-premises analytics systems.
Yalantis has experience working with leading BDAaaS providers powered by Amazon Web Services (AWS), Microsoft Azure, and Google Cloud Platform (GCP) to deliver comprehensive big data analytics solutions.
For example, in a recent project, we used AWS BDAaaS offerings to help a retail company analyze vast amounts of data from various sources, including point-of-sale systems, online transactions, and customer behavior data. Our data scientists and analysts used the following AWS services:
- Amazon EMR for large-scale data processing
- Amazon SageMaker for machine learning and predictive modeling
- Amazon QuickSight for data visualization and business intelligence
As a result, the client received a custom big data analytics platform that provided deep insights into customer behavior and sales trends.
Here’s a breakdown of services and benefits we offer when partnering with BDAaaS providers for big data analytics projects:
- Comprehensive analytics solutions: We use BDAaaS providers to deliver end-to-end analytics services, from data ingestion and processing to advanced analytics and visualization.
- Scalability and performance: BDAaaS solutions can handle massive datasets and complex analytics tasks, scaling seamlessly as your data and analytics needs grow.
- Cost-effectiveness: By using cloud-based services, BDAaaS eliminates the need for large upfront investments in analytics infrastructure and software.
- Advanced analytics capabilities: We integrate cutting-edge machine learning solutions and AI technologies provided by BDAaaS platforms to extract deeper insights from your data.
- Expertise and support: Our data scientists and analysts work with you to implement the most relevant BDAaaS tools and techniques, ensuring you maximize the value of your big data analytics initiatives.
Contact us
got it!
Keep an eye on your inbox. We’ll be in touch shortly Meanwhile, you can explore our hottest case studies and read client feedback on Clutch.
See Yalantis reviews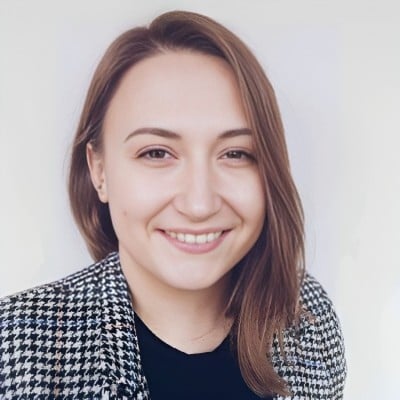
Lisa Panchenko
Senior Engagement Manager
Your steps with Yalantis
-
Schedule a call
-
We collect your requirements
-
We offer a solution
-
We succeed together!