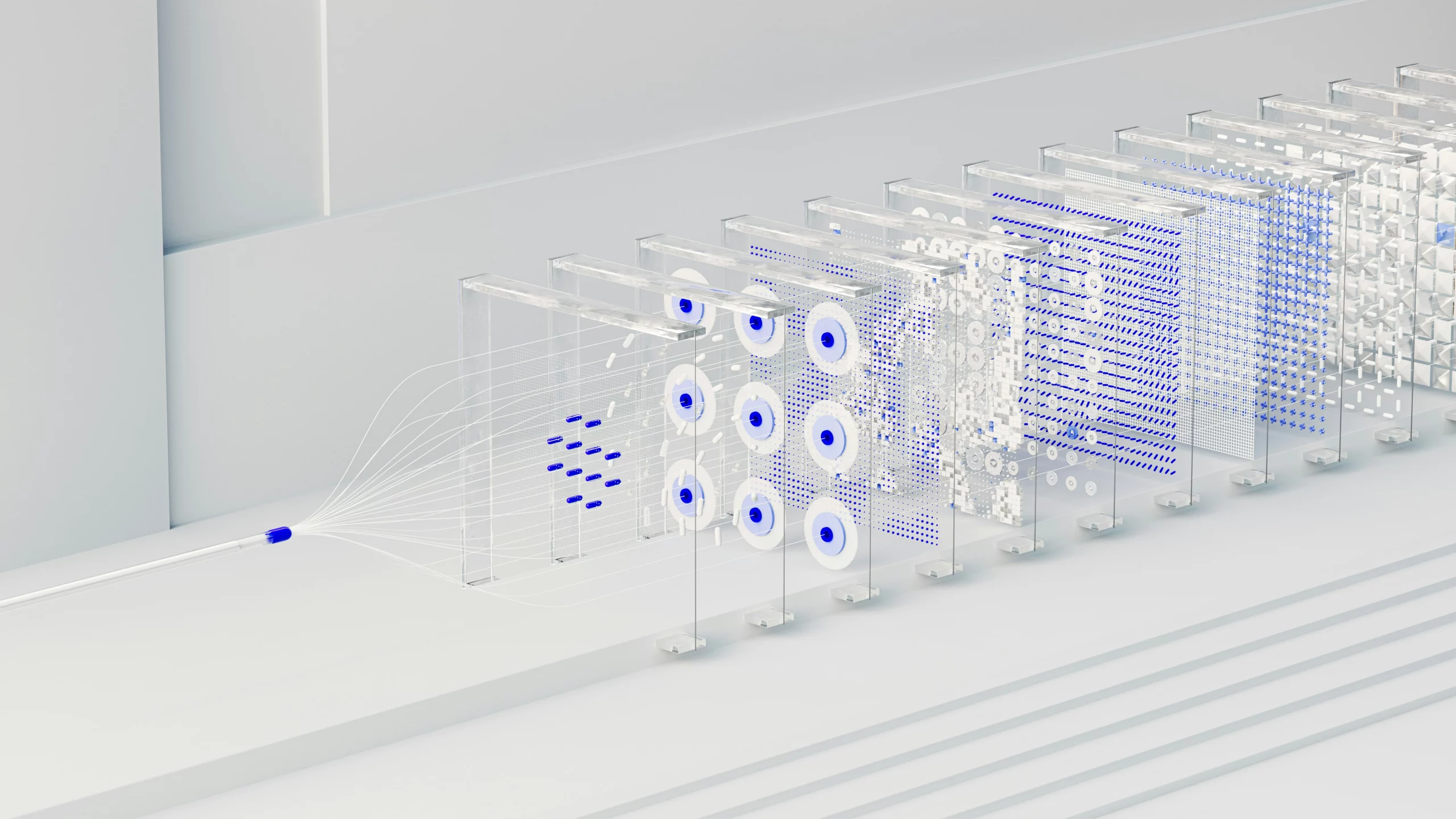
Machine learning use cases and potential value of ML technology across industries
This isn’t yet another article capitalizing on the AI and ML hype. We won’t bombard you with AI benefits that are irrelevant for your business or simply convince you to follow all the trends. Instead, let’s take it slow and see how you can implement machine learning (ML) on top of your existing data analytics tools.
“Data is the new precious metal of the 21st century. If you, as a business representative, know how to ensure high data quality and effectively utilize your data using ML and AI, then you can get it all: a competitive advantage, automation, increased business efficiency, and much more.”
Denys Doronin, Chief Technology Officer at Yalantis
A 2023 Rackspace Technology survey among 1420 IT professionals worldwide reveals that nearly 33 percent only just began their AI and ML journey in the past year, compared to 17 percent who began their AI and ML initiatives two years ago and 11 percent who started three years ago. These findings emphasize that businesses across diverse industries see the value in AI and ML adoption and are ready to implement cutting-edge technologies.
What drives current enterprises to integrate ML algorithms? This article guides you through reasons for ML adoption and machine learning use cases that promise tangible value no matter what industry you operate in. You’ll get a detailed checklist to see whether you’re ready to adopt ML immediately or perhaps need some time to prepare. Let’s take the first step into this swirling AI world by defining the value of machine learning.
Discover how to drive business value with AI and avoid expensive missteps
Explore our white paperWhat ML brings to the table for modern businesses
Suppose data analytics practices such as business intelligence (BI) are already a part of your business workflow and play a significant role in decision-making. In that case, machine learning development could be a winning option. Data science and, in particular, machine learning development is the next logical step after implementing BI, as it helps you dive deeper into data analysis, shifting from gathering, sorting, and analyzing the data your business generates to making justified business predictions based on BI-powered insights. Data analytics services can further support this transition by offering specialized tools and expertise to unlock valuable insights from your data. This includes processing large amounts of data, forecasting business performance, and even receiving suggestions for improving your business processes.
BI works well for defining and analyzing your business’s past and as-is state, but ML gives you the power to predict the future.
Explore our ML servicesAI models can learn from your business data by themselves and automatically perform routine tasks such as:
- answering complaint emails
- defining fraudulent payment activities or anomalies in the production line
- notifying you about all issues right away
These and many more features make machine learning services and data science services a strong opportunity for businesses to enforce progressive business strategies, adopt a growth mindset, and maximize the potential of their treasure trove of data.
How different businesses justify their choice of ML solutions
According to the 2023 Rackspace Technology survey we mentioned at the beginning of this article, here are the top five reasons why businesses choose ML:
Improve speed and efficiency of existing business processes. Machine learning can be integrated into multiple business processes simultaneously to improve overall business efficiency. For example, ML can define the root causes of recurring problems or classify customer concerns and complaints to help support teams quickly deal with them.
Predict business performance and industry trends. Machine learning is a go-to option if you want to know what the future holds for your business. ML uses mathematical formulas and statistical methods and integrates with third-party services to accurately predict future outcomes based on industry trends, market fluctuations, and past and current business processes. For instance, ML models can be trained to predict how new products and services will be accepted by the target audience in the current industry landscape and what ROI you can expect.
Reduce business risk by analyzing simulated situations. With ML, you can also predict and track anomalies and issues to better prepare for solving or even preventing them in the future. Machine learning enables you to perform risk-free experiments with synthetic data and validate your most ambitious ideas. ML can also assist you in improving your business strategy: for instance, by evaluating different countries to indicate where you might most successfully expand your business. To make such ML-driven insights truly effective, businesses often rely on robust big data development services that ensure accurate data aggregation and processing at scale.
Enhance personalization in the work environment. Apart from performing regular employee satisfaction surveys, you can use ML algorithms to learn more about your employees’ performance and preferences. It’s not about spying on your employees, but rather the opposite: an opportunity to connect and show them that you’re attentive to their needs and opinions.
In 2019, The Most Loved Workplace Research Team surveyed 3,600 employees in different spheres about their emotions and then created a custom ML algorithm to automatically analyze feedback and extract insights by breaking down feedback by sentiment and key topic. Thanks to this approach, researchers could quickly define the emotional connectedness of employees to their workplaces and form a framework that executives can use to improve their relationship with in-house teams.
Offer new or alter existing services. By analyzing large datasets, especially with unsupervised learning methods, ML models can help you see patterns and correlations in your datasets that business intelligence services aren’t capable of showing.
“The reasoning behind ML adoption boils down to innovation and speed. Two criteria that can help your business survive in the current economic climate with customer demands constantly shifting regardless of the business domain.”
Denys Doronin, Chief Technology Officer at Yalantis
ML use cases across industries: Each year marks more followers
Machine learning is rapidly proliferating among various industries and companies of different sizes, automating complex tasks and unburdening humans from work that could take them years to complete. But each machine learning use case is different, let’s find out why in this section.
“Given a limited number of resources, small businesses can benefit from launching a niche AI or ML solution to win the market. Midsize businesses may already have many interconnected systems and lots of datasets, and they can use AI or ML for scaling. Large enterprises, in turn, can capitalize on diverse AI/ML use cases to improve their operations at the departmental level.”
Denys Doronin, Chief Technology Officer at Yalantis
Statista has conducted thorough market research to define an approximate percentage of companies in different domains that have already implemented ML to indicate which industries were taking the lead in this technology as of the last update in August 2023.
Below is detailed coverage of use cases for machine learning in four industries: manufacturing, financial services, healthcare, and real estate. The first three industries are the most eager ML advocates according to Statista, but we’ve also added real estate to the list (even though Statista didn’t mention it), as we’re experienced in delivering advanced real estate software solutions and the real estate industry is also rapidly adopting machine learning technology.
Manufacturing use cases for machine learning: Ensuring streamlined and productive assembly lines
- Detect anomalies. Unexpected equipment failure, sudden electricity outages, and incorrectly assembled products cost the manufacturing industry lots of money. According to the pie chart above, manufacturing is the leader in ML adoption (with 18.88% of manufacturers having adopted ML as of 2023), and that’s mainly because ML models can help manufacturers predict and tackle lots of issues in a timely manner, reducing risk.
- Automatically adapt equipment to production and processing requirements. Machine learning is also capable of automatically programming production equipment. It can help manufacturers better control their assembly lines and reduce expenses from production line failure.
Real-life machine learning use case examples:
Bosch has set an ambitious goal of manufacturing every product either with the help of AI or fully AI by 2025. They’re also actively using AI and ML to detect anomalies or bottlenecks in their manufacturing process at the early stages and efficiently define root causes to learn from every failure.
Machine learning use case examples in finance: Benefitting from ML to facilitate business growth
- Offer personalized financial services. ML algorithms can analyze vast amounts of customer behavior data and define personalized options to increase customer loyalty. For instance, with the help of ML, a digital banking application can gather information about a customer’s most frequent purchases throughout a given month and then offer personalized cashback services from retailers of products that may be of interest to the customer.
- Improve credit approval decision-making. ML can allow financial organizations to automate and speed up credit approvals so that customers can take loans within minutes and without time-consuming paperwork. From the business perspective, ML algorithms can analyze customer data to quickly define customer credibility for loan issuing.
- Ensure accurate fraud detection. Data privacy and security are essential for financial institutions, and ML can be a helpful assistant in this. Custom ML algorithms can be trained to effortlessly detect fraudulent activities by filtering large sets of customer financial data.
Real-life examples:
#1: Apart from powering their AI assistant, Wells Fargo uses AI and ML as the backbone for developing a data science platform that will help their internal data science team efficiently develop AI solutions to further enhance the customer experience.
#2: Standard Chartered Bank uses ML for automating manual business processes, detecting fraud, and providing customers with personalized communication approaches, offers, and services.
Prominent machine learning use case in healthcare: Applying ML to enhance the patient experience and unburden medical staff
- Advance precision medicine. ML algorithms can be used to predict drug responses for high-risk patients and compose individual treatment plans based on laboratory analysis and lifestyle habits. With ML, it’s also possible to facilitate clinical decisions and help clinicians make accurate diagnoses faster. This is significant, as winning time in disease treatment can be life-saving.
- Analyze medical images. This ML use case has proven extremely efficient in medical image analysis, as it can predict serious illnesses such as cancer and cardiovascular diseases and allow patients to start treatment at early stages.
Real-life examples:
Medical researchers at Cambridge University have tested a machine learning tool that can analyze brain MRI scans and predict the onset of Alzheimer’s disease before the first symptoms occur. This allows clinicians to prescribe medication beforehand and give patients lifestyle tips that can help delay the onset and progression of the disease.
Find out how we developed a cloud-based medical imaging system for a distributed hospital network.
Learn moreReal-estate ML use cases: Enterprises can make good use of ML to increase profits and stand out in the market
- Evaluate property conditions. ML models can be trained to go through a wide selection of images for different properties, define each property’s condition, and compose a ranking system. This way, buyers and sellers can avoid bias in property analysis and get an all-around report covering a property’s true condition as well as price suggestions.
- Provide personalized property and rent recommendations. Based on search results, ML models can offer consumers options similar to what they’re interested in buying or renting. This way, potential customers may stay on the website longer and are more likely to make a purchasing decision.
- Define property investment risk. By considering multiple factors such as market conditions and a consumer’s financial situation, ML algorithms can define a risk score for property purchasing and even provide investment advice. Potential risks can be associated with the economic condition of the city or country, fluctuations in loan interest rates, and even potential environmental risks.
Real-life examples:
#1: A wide selection of Zillow features are based on machine learning, such as personalized home recommendations, generation of building floor plans, and semantic search capabilities.
#2: HouseCanary employs ML for unbiased assessments of a home’s condition, value, and quality. Plus, machine learning models identify variables that affect house price formation the most, allowing HouseCanary to put the most emphasis on them and, for instance, prioritize them in their property filtering system to simplify property searches.
Learn about machine learning algorithms for predicting home prices.
Read the articleFor each business case, there can be individual machine learning applications that can differ from those we’ve mentioned. The most crucial success factor of ML implementation is carefully thinking through a problem to solve with ML. In the next section, you’ll learn more about this and what else is necessary for fruitful ML application.
Find out whether your company infrastructure is ready for ML implementation.
Download a free checklistYalantis’ approach to running successful machine learning projects: machine learning techniques and implementation steps
Machine learning algorithms can perform two major tasks with your data: classification and prediction. Depending on whether you feed ML models with labeled or unlabeled data, machine learning algorithms can be divided into supervised and unsupervised. Apart from that, there is also reinforcement learning, which suits a limited number of machine learning business use cases such as in the gaming industry and robotics and involves training a machine learning model through a sequence of errors and successes, with the model learning better how to avoid errors the more it makes them.
Labeled data refers to datasets with certain characteristics (for example, medical images such as X-rays, MRI scans, or CT scans that have labels indicating areas of interest like tumors, fractures, or other abnormalities). Such datasets are used for training supervised machine learning models. Unlabeled data, in turn, refers to datasets without any characteristics (for example, time-series data from IoT devices and sensors installed at manufacturing plants). Unlabeled data is suited for training an unsupervised machine learning algorithm.
“Businesses may have data with which they want to teach an algorithm to solve certain practical tasks. But it may turn out that the data is labeled incorrectly or is too specific. That’s why we need to carry out a feasibility study to define if the collected data is suitable for implementing an ML model.”
Serhii Zhuravel, Director of Engineering
Supervised and unsupervised machine learning have different goals and techniques. With supervised learning, you need to have a clear problem for an ML model to solve. For unsupervised learning, your goal can be to define new patterns by analyzing diverse unlabeled datasets. Unsupervised machine learning can be compared to a brainstorming session with all of your team members to define new solutions to old problems. Supervised learning is similar to a board meeting, with each executive analyzing documents with clearly defined business outcomes.
Supervised machine learning algorithms:
- Classification. This ML algorithm categorizes labeled datasets based into predefined classes. The classification ML approach requires a large amount of training data to feed ML algorithms and create high-functioning ML models. Classification works well for differentiating between legitimate and spam emails, or classifying images by class (such as animal species, product groups, car models, and professions). Typical classification models are logistic regression, decision trees, Naïve Bayes, K-nearest neighbors (KNN), and random forest.
- Regression. This is another approach to supervised learning that involves predicting future outcomes based on previous data, such as predicting house prices based on previous prices for similar properties. Another example could be predicting a building’s energy consumption, taking into account multiple variables such as the number of apartments and the approximate number of electrical appliances used in each household. Typical regression models are time series models, gradient boosting machines (GBM) for regression, ridge regression (L2 regularization), lasso regression (L1 regularization), and elastic net regression.
Unsupervised machine learning algorithms:
- Clustering. A clustering machine learning algorithm separates large chunks of unlabeled data into clusters of datasets with similar parameters. Unlike classification algorithms, clustering ML algorithms need neither training data nor predefined clusters to separate data. An example of clustering can be analyzing different city districts and defining neighborhoods with the most convenient infrastructure and highest lifestyle quality. However, it’s advisable to validate the outputs of clustering algorithms, as they can group the data in clusters that make no sense to your business and don’t provide valid insights. Typical clustering algorithms include K-means clustering, hierarchical clustering, density-based spatial clustering of applications with noise (DBSCAN), gaussian mixture models (GMM), agglomerative nesting (AGNES), and density-based clustering extensions
Classification, regression, and clustering can be further divided into diverse techniques that help solve various classification or prediction tasks. There is an immense number of ML models, some of which may not even fall under the classifications we’ve provided in this section. But any information is better understood when it’s structured at least to a certain extent. Defining the right approach to training an ML model that solves your particular business problem or helps achieve a certain goal is an intricate task that requires a skilled data team to thoroughly prepare your data infrastructure. Applying AI development services can ensure that these processes are executed efficiently and effectively.
Learn about key aspects of data lifecycle management that play a crucial role in efficient data analysis.
Read the articleHow to integrate ML solutions and avoid workflow disruption and people resistance: Yalantis has a four-step plan
As we’ve done with all other technologies we’ve integrated during our 15-year presence in the IT market, we’ve developed a structured approach to ML implementation. It consists of four steps.
Step 1. Begin with a simple goal or problem
To avoid an abrupt disruption of your business workflow, you should take ML adoption slowly — especially if machine learning is a new undertaking for your organization. Set a simple and realistic goal to solve a certain problem, such as defining the reason behind customer churn or why buyers abandon their carts during the holiday season. Or you can choose to anticipate your customers’ future needs with ML and experiment by offering discounts on certain services or perks such as free shipping.
“ML comprises algorithms fed with structured data to perform tasks that aren’t rule-based with much higher accuracy than humans. When working on ML projects, we first need to clearly define the problem to solve. Only then can we integrate a suitable ML model, refine it if the initial solution was incorrect, and monitor the model for consistently correct results.”
Serhii Zhuravel, Director of Engineering
Step 2. Build a proof of concept
To stay on the safe side, you can solve the problem or achieve the goal you’ve identified in step one by first developing a proof of concept. This way, you can avoid any risks and examine first-hand the value of ML for your business.
Step 3. Evaluate the results
Once you have a proof of concept, you can measure its results. And if your attempt fails, you can learn from it even more, as you’ll be able to prepare much better for the next attempt and have a higher chance of succeeding. Your PoC can already be helpful by indicating why you couldn’t solve the problem or achieve your goal using other methods. In addition, the biggest benefit of ML algorithms is that they help you avoid bias, as they don’t pursue personal goals, unlike stakeholders who can have hidden subjective reasons for making certain business decisions.
Step 4. Fearlessly expand
By no means should you stop at the PoC stage. Rather, you should expand and scale your ML solution by feeding it with more data from a greater variety of sources, such as IoT devices and third-party services. You can conduct risk assessment sessions and involve more stakeholders and data specialists to increase your confidence and progress further with machine learning projects. At this step, you can modify your initial problem or goal and choose a more complex one.
In between these four steps, there is a lot of technical work that involves collecting and preparing data and choosing machine learning technologies and algorithms that would work best for your particular problem. We’ve generalized the steps on purpose so that you as a business leader can see ML implementation entirely from your perspective. Being brave and ready to take calculated risks is the key to success with such a disruptive and powerful technology as machine learning.
Once implemented, ML will completely transform your decision-making, help you quickly identify and solve business blockers, and increase your business efficiency as well as speed of service delivery. And the faster and more innovative you are in these uncertain times, the better the chance that your business remains profitable and competitive. Yalantis’ data and ML engineers can become trusted partners on your way to a better-organized and more insightful future.
Automate and optimize your business workflows with ML algorithms
Collaborate with Yalantis ML team
Contact usFAQ
What programming languages and frameworks does your team specialize in for ML development?
For machine learning solutions and also diverse AI/ML use cases, we employ the Python, R, and Java programming languages. A wide variety of packaged ML algorithms are available via APIs such as TensorFlow, Spark MLlib, Hadoop, Airflow, and H2O. We also use machine learning as a service (MLaaS) tools offered by Amazon, Microsoft, and Google.
What methods do you use for training and evaluating ML models?
Our data scientists and ML specialists have experience in supervised, unsupervised, and reinforcement learning, which we apply depending on different use cases of machine learning. To evaluate model performance, we use metrics such as precision, accuracy, recall (sensitivity), F1 score, specificity, and receiver operating characteristic curve (ROC).
Is there a chance that artificial intelligence and machine learning can be dangerous for the business community and society?
ML is an amazing opportunity for the business world to make many people’s lives easier, healthier, and happier. To our mind, that’s the only purpose it should be used for. Thus, it’s essential to use machine learning responsibly, follow ethical rules, and comply with emerging laws and regulations.
Rate this article
4.8/5.0
based on 57 reviews