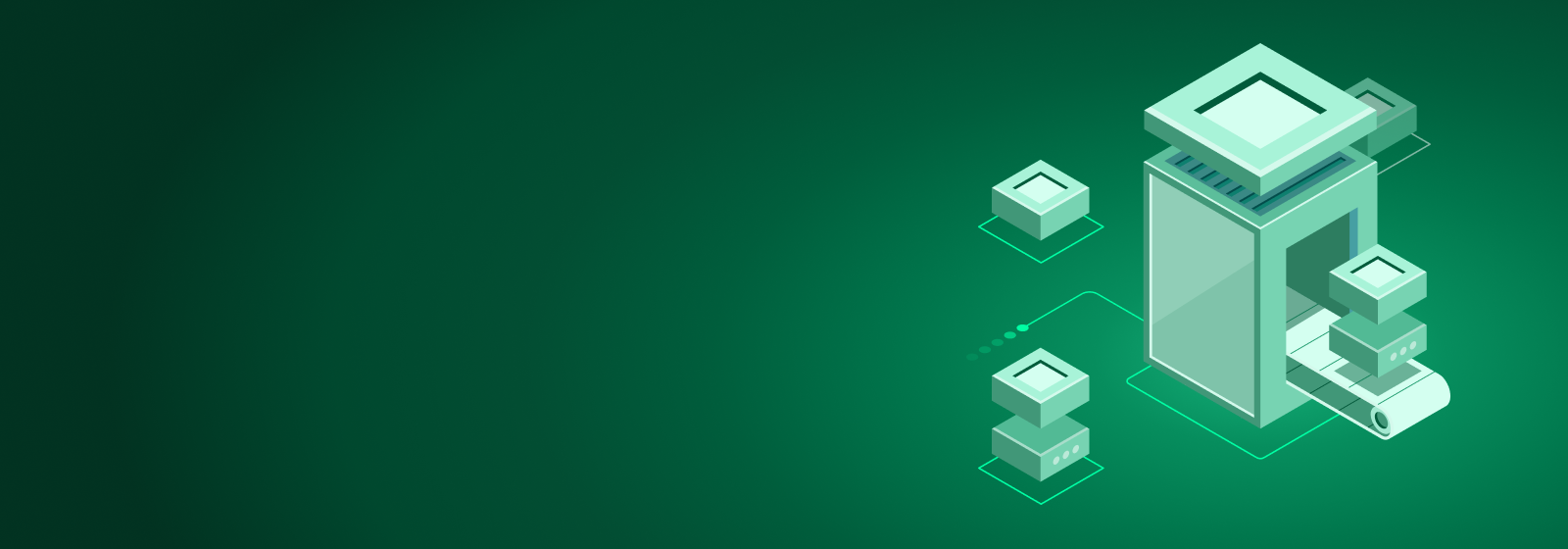
Instant analysis of time-critical data: Real-time data streaming use cases, examples, and benefits
It’s true that for a well-functioning data streaming process, you need to invest in a complex data infrastructure, and depending on the data collection frequency, costs can differ. For instance, 71 percent of executives (according to a survey of 300 decision-makers in data management across industries) frequently experience “bill shock” when receiving payments for maintaining data infrastructure.
But what if we told you that there is a cost-efficient data streaming solution for everyone? To match unique business needs, we choose a unique mix of technologies and services to provide optimal and, most importantly, customizable data streaming software that naturally scales with your business.
The benefits are worth it. Tapping into real-time streaming data helps mature businesses—established companies collaborating with many clients and generating large volumes of data—drive innovations, improve customer experiences, and fuel their digital transformation initiatives. How is that possible? With a perfect blend of data technologies, experienced data engineers, and cross-company change management strategies.
Let’s dig deeper into why, what, and how of data stream processing. In this article, we cover data streaming use cases, real-time data streaming examples, the benefits of implementing data streaming solutions, and find out what’s under the hood of such software.
Data streaming: Rewarding path from data silos to real-time insights
Real-time data streaming is the process of instant data collection and delivery. Unlike batch processing, which operates on historical data and can gather information over time, with real-time stream processing, you can quickly get the data from various sources, analyze it, and act on it to fulfill different business needs or goals. A 2024 data streaming report—composed by Confluent based on insights from 4,110 IT leaders—reveals that:
- 79 percent consider data streaming platforms (DSPs) crucial for business agility
- 63 percent view them as an accelerator for AI adoption
- 93 percent are convinced that these platforms help them overcome obstacles such as data silos
Efficient data management is the main fuel of real-time data stream processing
The abovementioned statistics prove that respondents perceive streaming data as a tool for enhancing their business operations. However, data will work to your business’s advantage only if it’s properly managed. For instance, besides a lack of finances, the most common reasons for failing machine learning projects are data issues:
Thus, to implement a real-time streaming data process that matches your evolving business initiatives and drives innovation, you first need a secure, scalable, and high-performance data infrastructure. Wouldn’t you want to be among those two percent with successful ML projects? We think it’s worth striving for.
What to expect after you adopt data streaming software
Let’s take a look at the before and after scenarios of the data stream software implementation.
There is a vast gap between businesses that adopt streaming data solutions and those that either lack this process or don’t have the capacity and understanding of how to organize stream processing for their businesses. Implementing batch processing can also be efficient, but it won’t give that on-time perception of your business operations.
To bridge the gap between these two worlds of siloed data and real-time insights, you’ll need to be prepared for a long but rewarding journey with the help of diverse data engineering services. In the next sections, we dive deeper into this journey’s benefits and technical aspects of stream data processing.
Real-time streaming data examples to derive insights from
The data that’s best for streaming falls under several categories:
Web or mobile clickstream data. When your clients interact with your products and services via a website, web application, or mobile application, you can immediately stream that data to visualize, analyze, create a report, or use it for data science projects. The frequency with which you can stream data depends on your needs. For instance, during Black Friday, an e-commerce company might need to know the exact number of purchases every few minutes to update stock information on a website.
IoT data. Process streaming data from IoT devices, sensors, and applications is also common. Such data, enhanced through AI development services, includes temperature readings, machinery conditions, health metrics, weather fluctuations, and consumer behavior information in stores. However, IoT data comes at a high speed and often in different formats. The challenge is transforming streaming data into the right format for data analytics or AI/ML models to run on, which can be efficiently handled by an IoT data analytics platform. As a big data services company, we specialize in processing and analyzing such high-velocity data to derive actionable insights in real-time, often leveraging advanced data science services to extract deeper insights from complex data streams.
Application and system logs. Customer-facing applications generate lots of data worth collecting via stream processing to provide customers with personalized services. For instance, a health monitoring app offers recommendations for improving lifestyle based on a patient’s health metrics, eating habits, and current medication intake. Internal applications and systems that your employees use can also be a valuable source of insights during stream processing. For example, you can gather real-time information from your customer support system and check whether your employees can efficiently handle clients’ issues.
Financial transactions are also a common data stream example, such data as credit card transactions and stock market fluctuations require immediate reaction and analysis to detect fraudulent activities and notify customers on time to avoid reputational and financial losses.
Social media data. Gathering real-time data from your social media accounts (comments, likes, reposts, trends, viral hashtags) is critical to maintaining your brand reputation and ensuring that you nip any problem in the bud if it occurs. On the contrary, if your social media data indicates that a certain product or service is a huge success—like those TikTok videos that fueled air fryer sales—you can use the hype to your advantage and increase sales.
From these streaming data examples, you can choose the data types that would be most important for fulfilling your current business goals. In fact, we advise you to always keep your goals in mind when deciding on adopting any technology.
Short- and long-term benefits of data streaming software for your business
You would think that data streaming offers only short-term benefits since it’s time-critical and relevant only for a short time. And then you would wonder whether it’s all worth it. If you miss the moment to analyze or visualize data in real time, then your complex and costly data infrastructure won’t even be necessary. The answer to your concerns is two-sided:
- Yes, streaming data is time-sensitive, but the insights you derive from data streams are relevant for much longer.
- Yes, it’s possible to miss the opportunity to analyze data streams, but you can set up a custom automated process for streaming data analysis to get timely insights.
Let’s discover both short- and long-term benefits of extracting insights from streaming data:
1. Decision-making that makes a difference and affects current and future business operations. Real-time insights help you make the right decisions. For instance, you can alter your services to match current customer and market needs better. And such a decision then impacts your overall business strategy. For example, a manufacturing organization can timely discover a malfunctioning product and decide to cease production, opening opportunities for new product ideas.
2. Strong customer relationships also have long-term effects on your organization, as satisfied customers are more likely to stay loyal to your company and become its ambassadors. Leveraging real-time customer behavior data and preferences can help a retailer improve their store layout, a hospital optimize patient rooms, and a digital bank offer convenient loan management. Collecting and analyzing real-time customer data can be quick, but acting on it can be prolonged to achieve maximum positive effects.
3. Optimized risk appetite. Continuous risk management can be a powerful tool for identifying and capitalizing on business opportunities while avoiding potentially unsafe judgments. Backed by real-time data, business owners can feel more confident making rewarding business decisions and avoid getting stuck in overanalyzing. Taming and optimizing streaming data makes a business more flexible and ready for change.
4. Increased sales and revenue should be mentioned, too, as when you start paying attention to real-time or near real-time company performance and have a proactive mindset, you start seeing ways of increasing sales and opening new revenue streams. That’s the power of insights during stream processing. They fuel your business growth.
Once we understand data streams and their potential benefits, we can move on to stream processing use cases—the practical side of applying all those insights to your business advantage.
Data streaming: Analytics use cases with real-life examples across industries
Extracting maximum value from data streams that come from various data sources is a complicated yet rewarding task. Here are a few typical use cases for stream processing:
#1. Real-time data analytics services. Instant stream data analysis allows businesses to gain insights into their company operations, customer experience, employee satisfaction, or any other important aspect. Insights derived from streaming data can feed a decision support system to improve decision-making accuracy and drive digital transformation initiatives.
For example, the Nova Scotia Health Authority has shifted from paper processes to real-time patient care across their 44 facilities by implementing various digital solutions and stream processing. They achieved a 91 percent increase in real-time data timeliness and accuracy to use for their decision support system and replace stale data reporting with automated near-time insights.
#2. Edge analytics. Streaming data coming from IoT devices and sensors can be a fuel for time-efficient edge analytics. A Belgian food manufacturer, Clarebout Potatoes, has adopted real-time edge analytics to meet the growing demands of their clientele across 60 countries. The company needs to maintain a continuous manufacturing flow and process data without interruptions and costly downtime, and a real-time scalable streaming data platform serves as a glue, uniting all their production data for timely anomaly and fraud detection. Thus, Clarebout Potatoes can quickly analyze streaming data in real-time close to the source to increase dexterity and serve customers without delay.
#3. Data science projects. AI/ML algorithms can be trained on streaming data for anomaly detection, and predictive and prescriptive analytics. A financial company, Capital One, is gaining an advantage from the real-time data streaming process by collecting real-time transactional data for stream processing and assessing risks to protect their customers.
Another example is healthcare technology company Medtronic, which gathers real-time surgical data to train 14 AI algorithms that help surgeons gain comprehensive post-procedure insights. The company has also launched remote secure live-streaming of surgical procedures to enhance healthcare training across diverse countries via efficient stream processing.
#4. Personalized marketing. Another common use case is collecting streaming data to provide consumers with individual marketing proposals. For example, Walmart has set up an end-to-end data streaming and processing flow to track every order to improve order fulfillment and increase customer satisfaction.
Streaming data used in combination with the right technology, such as AI, ML, or business intelligence, is a powerful foundation for a resilient and customer-oriented business model. In the next sections, we discover what it takes to implement a comprehensive data stream solution and set up functioning stream processing.
Mechanics of the data streaming implementation process: 6 success rules from Yalantis’ data and BI engineer
Vladyslav Mysholovskyi, our data and BI expert, has developed a 6–rule framework that can help businesses enforce a well-functioning real-time data streaming and data processing system:
Rule 1. Establish a high-performance infrastructure. Your data infrastructure needs to handle massive amounts of data streams generated continuously from many sources. Since data flows in real time, systems have to scale efficiently without losing performance.
Rule 2. Mind data consistency and accuracy. Inconsistent data stream processing can lead to incorrect conclusions and poor decision-making. This challenge can be addressed by implementing robust data governance practices, which include data validation, cleansing, and transformation processes. Using extract, transform, load (ETL) tools can automate these processes, ensuring that data is standardized and accurate before you use it for analytics.
Rule 3. Ensure low latency to enable real-time analytics. A company should employ advanced technologies and efficient systems for instant stream processing and insight delivery. For example, in-memory computing frameworks like Apache Spark allow for rapid data processing, while edge computing devices enable data analysis closer to the data source, reducing latency.
Real-time data streaming platforms like Apache Kafka and cloud services like AWS Kinesis provide the necessary infrastructure to handle and process data with minimal delay. Plus, such systems provide a reliable and balanced separation between data producers and consumers, ensuring better scalability and flexibility of the data processing and transferring.
Rule 4. Develop workarounds to integrate with legacy systems. Integrating legacy software with modern analytics tools can be complicated and time-consuming, often requiring significant modifications and innovative solutions. Several strategies can be employed to address the integration challenge of outdated systems with data streams and modern analytics tools:
- Gradually migrate data and functionality from legacy systems to modern platforms, ensuring compatibility and minimizing disruption to operations.
- Employing middleware solutions that act as a bridge between old and new systems can facilitate smoother integration by translating data formats and protocols for effective data processing.
- Leveraging cloud-based integration platforms can provide scalability and flexibility, allowing businesses to adapt and upgrade their data stream systems incrementally while maintaining functionality and enhancing analytics capabilities.
Rule 5. Clean and prepare raw data in real time to ensure high-quality stream processing and inputs for analytics. Raw data often contains errors, duplication, and irrelevant information. Tools such as Apache Spark can automate the data cleaning process, while best practices like data validation, deduplication, and the use of data quality frameworks help maintain accuracy. Microsoft Azure Data Factory and Google Cloud Dataflow also offer integrated solutions for real-time data transformation and quality management, ensuring that the data used for real-time analytics is reliable and actionable.
Rule 6. Be realistic and remember that combining data from various sources requires complex tools and processes. To achieve efficient data stream processing, businesses can use ETL approaches to ensure that data is accurately extracted, cleaned, and transformed before being loaded into a unified data warehouse or data lake, such as those offered by Google BigQuery or Amazon Redshift. This approach ensures seamless data integration and consistency across the entire organization.
Common tools and technologies for streaming data platform development
Below is a modern data streaming architecture from AWS documentation to clarify how real-time data streaming tools combine into a functional data architecture.
Stream sources we discussed earlier in the article, so let’s focus more on data streaming and data processing tools and technologies during stream ingestion, storage, processing, and outcome (or the insight generation process). The exact choice of these tools differs depending on a business use case. Here are the most essential:
Streaming data ingestion:
- Real-time data integration platforms (Apache Kafka, Apache Flink, Google Cloud Dataflow, AWS Kinesis). These platforms enable the real-time integration of data from various sources, ensuring synchronization and consistency. They play a key role in creating a unified analytical environment.
Streaming data storage and stream processing:
- Cloud platforms (AWS, GCP, Azure Solutions). Cloud platforms provide a scalable and flexible infrastructure for storing and processing real-time data. They allow businesses to quickly adapt to changing business requirements and ensure high performance and data availability.
- Database management systems (Google BigQuery, Amazon Redshift, Snowflake, Databricks). High-performance DBMSs are necessary for storing and quickly processing large volumes of data streams. They ensure reliable storage and access to data, which is critical for real-time analytics.
Insight generation:
- Business intelligence (BI) tools (Tableau, Power BI, Looker). These tools allow for data visualization and report creation, helping businesses make informed decisions. They support integration with various data sources and provide an intuitive interface for data analysis.
- Artificial intelligence (AI) and machine learning (ML) enable data analysts to analyze large volumes of data streams and make predictions based on identified patterns. ML model development services assist businesses in creating customized machine learning models that can predict trends, optimize decision-making processes, and provide deep insights for future strategies. AI/ML models help businesses personalize customer offers, optimize business operations, and meet market demands.
To create a unique data stream processing solution and choose the right combination of advanced technologies, you’ll need a skilled data engineering company with a proactive mindset that helps them make technical decisions that match your business goals.
Final thoughts: Taming continuous data streams to your business advantage
The main value of implementing a data streaming platform is that it allows your organization to keep up with the growing demands and expectations of your customers and stakeholders. It helps you remain afloat, always on, and on-brand. And the more your business capabilities align with customer needs, the more successful you’ll become.
This article began by exploring whether investing in a real-time data streaming platform is worthwhile. We hope it has provided valuable insights to help you make the right decision. However, you can request our consulting services if you need more information or personalized guidance to make the final choice. Yalantis will support your every step toward building a data-centric organization.
FAQ
What is a data streaming platform?
A data streaming platform combines advanced tools and technologies that enable real-time data collection from diverse sources, secure data storage and processing, and real-time data analysis. It provides businesses with insights that enhance their decision-making frameworks.
How do you help build a reliable data infrastructure?
To build an efficient data infrastructure, we prioritize data quality, security, and seamless integration with existing systems. Without well-organized data management processes, the potential benefits of real-time insights may remain concealed.
Why do we need a data streaming solution?
A data streaming solution can be helpful if you want to make sense of large volumes of real-time data. Such a solution provides real-time or near real-time insights that allow you to adjust current operations or services.
Rate this article
4.8/5.0
based on 18 reviews