The rise of generative AI tools has spurred lots of debate concerning whether artificial intelligence (AI) technology can be fully trusted to perform tasks instead of humans or whether it should be used only under strict human supervision. At Yalantis, we believe it depends on the type of task, as some work such as answering customer support queries or improving image quality can be easily entrusted to AI while other tasks that include working with sensitive data, such as compiling company policies, may require human supervision. Despite all the debates and ethical considerations, generative AI is gaining ground and opening lots of opportunities for business growth.
An Accenture Technology Vision 2023 survey among 4,777 C-suite executives across 25 industries revealed that 96 percent are inspired by the potential benefits of generative AI. For the generative AI domain, 2023 has been one of the most successful years so far. According to CB Insights, funding for the development of generative AI has reached a staggering $14.1 billion in 2023, compared to $2.5 billion in 2022.
Such figures aren’t surprising, as this subfield of artificial intelligence offers the opportunity to:
- expand a service offering
- come up with novel business solutions
- quickly meet shifting customer needs
- perform complex computational tasks
- enhance expertise and save resources without hiring and onboarding new employees
- unburden employees from performing trivial tasks such as daily customer support
Let’s explore the benefits and use cases of generative AI for different industries and find out how to prepare for integrating a generative AI model into your business workflow.
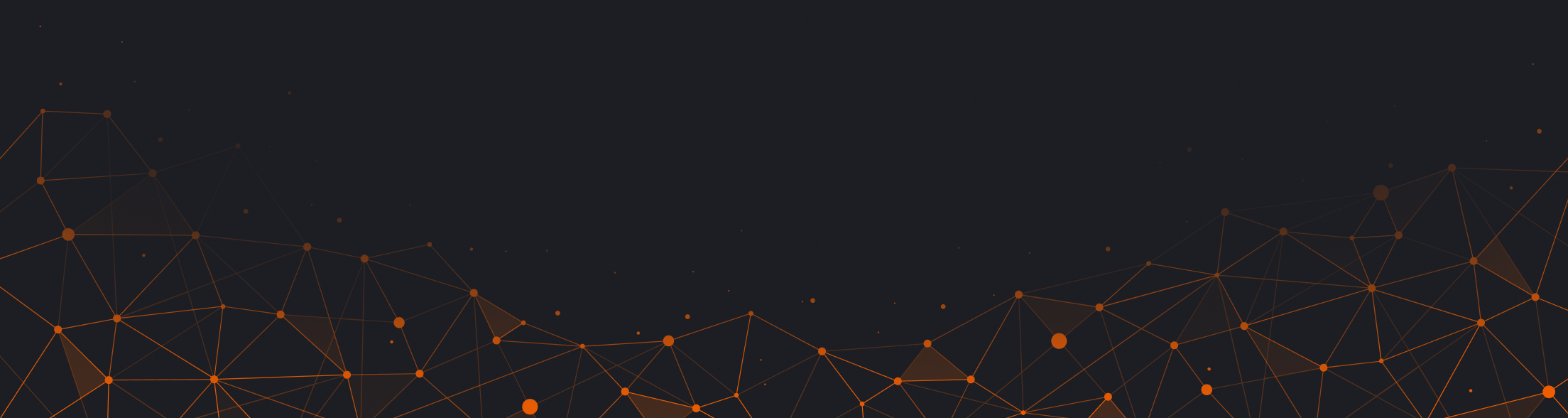
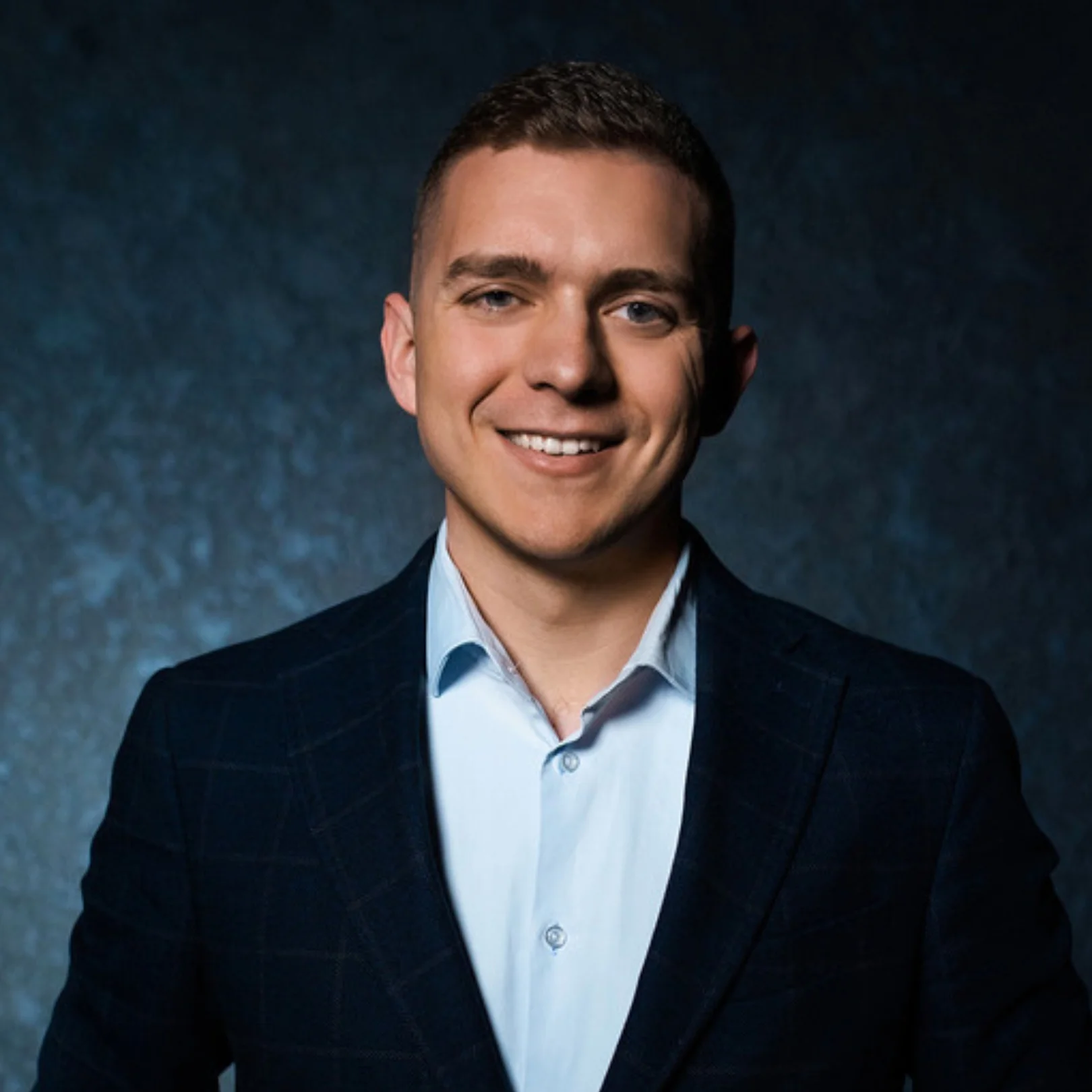
Discover how to drive business value with AI and avoid expensive missteps
Explore our white paperGenerative AI in a nutshell: what it is, how it works, and how it differs from other AI models
Section contents:
- Explanation of foundation models
- Difference between foundation and traditional AI models
- Working principle of generative AI algorithms
- Generative AI examples
Generative AI encompasses a wide variety of extensively trained models that researchers from Stanford University have named foundation models. Foundation models are large machine learning models trained using self-supervised learning at scale (use cases of machine learning). The difference between foundation models and traditional AI models lies in the fact that foundation models can be trained on a vast variety of data types such as text, images, audio, and video. Traditional AI models are more rules-based, task-specific, and dependent on human programming. These models can be trained on much less data than foundation models. However, traditional models are useful for efficiently performing repetitive tasks and receiving predictable and transparent results thanks to their rules-based nature. Both types of models (foundation and traditional) fall within the scope of Yalantis’ AI development services.
Foundation models such as ChatGPT and DALL-E 2 can easily adapt to different generative AI use cases and tasks, which can include:
- expertly answering questions
- creating unique images and improving image quality
- conducting sentiment analysis by analyzing millions of customer reviews
- defining customer satisfaction with business services
- creating lots of personalized content
Generative AI has huge power and potential to disrupt the common business workflow, allowing organizations to use high computational power and huge datasets to create completely new concepts, products, and services.
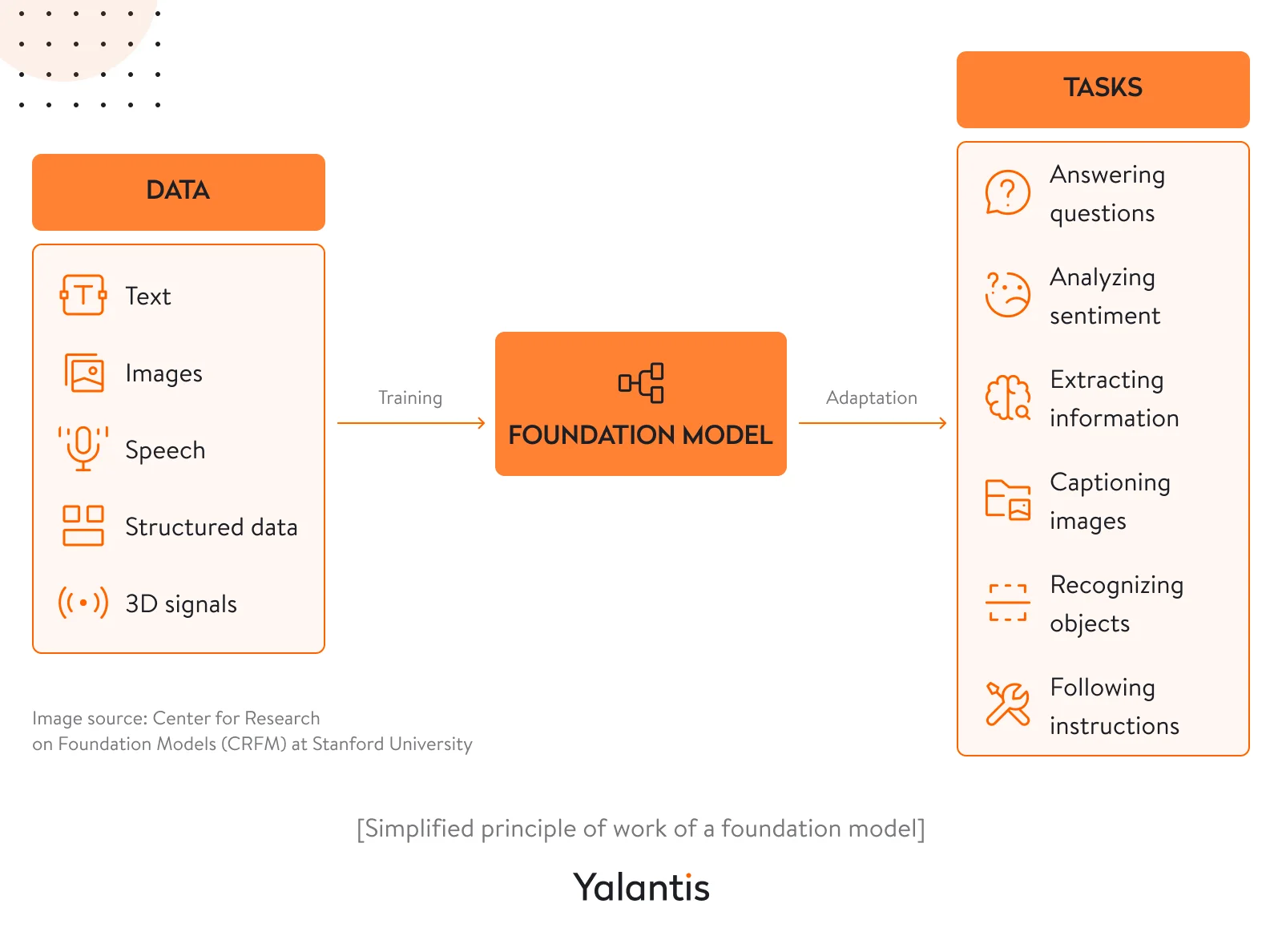
Under the hood, foundation models work based on diverse neural networks such as generative adversarial networks (GANs) that encode and decode natural language prompts and then output results. For instance, OpenAI uses a Contrastive Language–Image Pre-training (CLIP) neural network in their solutions to create images based on text descriptions. The schematic below illustrates how the CLIP neural network works and which components it includes to produce accurate results.
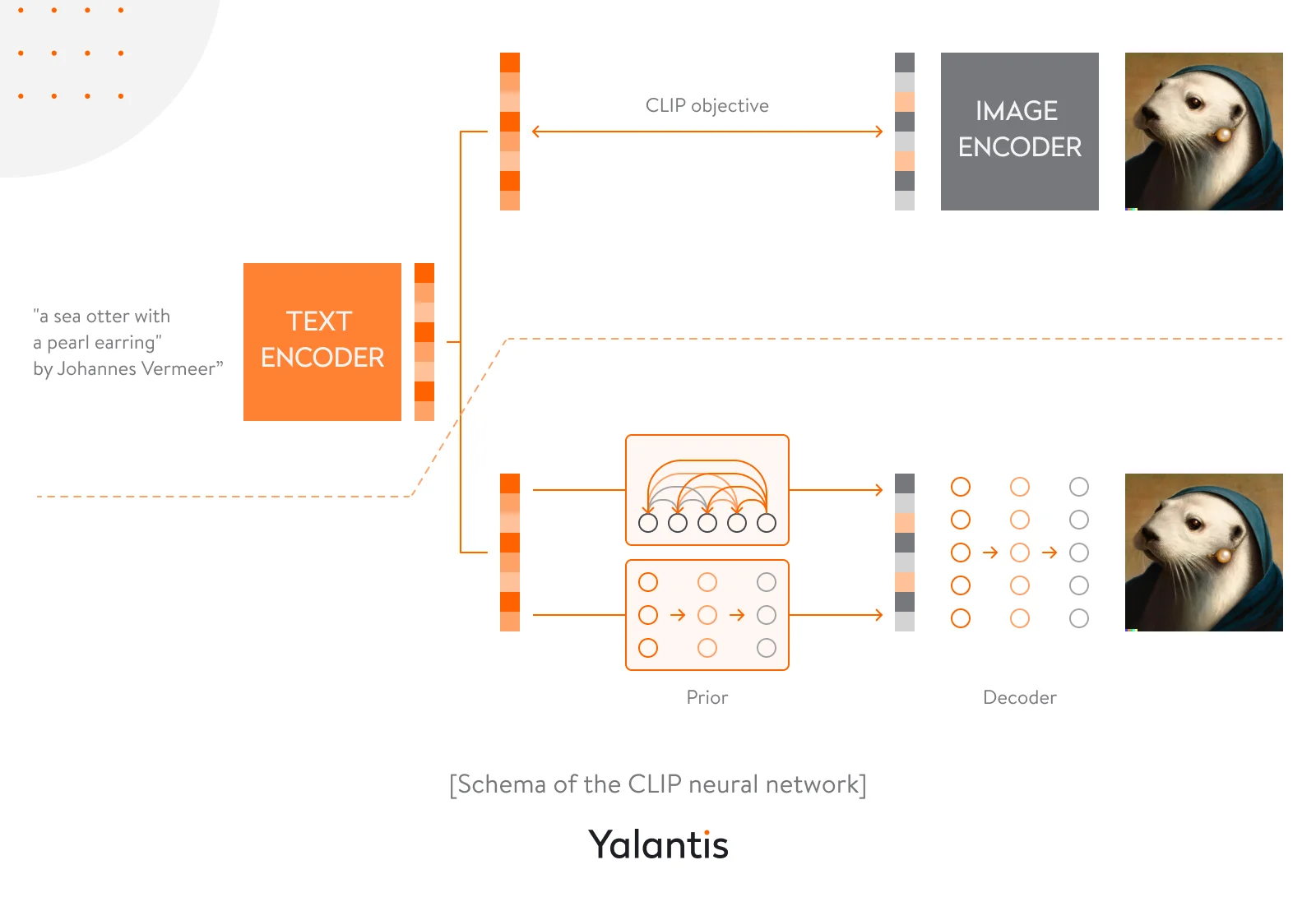
With a general understanding of what generative AI is, we can discuss the projected impact of this technology on the business world and the global economy. Within the scope of our generative AI software development services, we can help you dive even deeper into generative AI and form a comprehensive vision of how to use this technology for your business.
Potential impact of generative AI on the global business landscape
Section contents:
- How generative AI is evolving and changing the way we conduct business
- How improved productivity with generative AI applications can advance the global economy
- Reasons to adopt generative AI
According to a Gartner report on tech trends that are shaping our future, generative AI is among those trends that are responsible for accelerating business growth. This technology is considered an engine for rapid innovation, as it draws on training data yet manages to create unique outputs.
Generative AI has the potential to move the whole AI industry forward at an accelerated pace, even as other AI subsets such as advanced analytics (advance analytics services), machine learning (mlaas), and deep learning are steadily evolving and finding wider adoption for a variety of use cases.
Generative AI models can allow humans to perform tasks and conduct research in minutes instead of the months or years it would take to do manually, suggesting that in the near future, we may witness more potential cures to various diseases, much quicker technical support, accelerated software product development, and an increased number of services, products, and solutions compared to what we have today, each personalized to our unique needs.
Generative AI can also increase global workforce productivity by helping employees perform their daily activities, spurring creativity and assisting in problem-solving.
We can expect AI systems to become not only our assistants but also equal partners, as AI models are evolving and with time can achieve almost human levels of creativity, logical reasoning, problem-solving, and social and emotional awareness.
Factors that encourage businesses to adopt generative AI
A 2023 survey by Statista among 4,500 US professionals in different fields revealed the share of employees using generative AI in the workplace:
- 37 percent of specialists working in marketing and advertising
- 35 percent of technology workers
- 30 percent of consulting specialists (machine learning consultants beyond)
- 15 percent of healthcare workers
Even though some industries are adopting generative AI more eagerly than others, this technology is confidently gaining popularity, opening new opportunities for businesses to thrive. Let’s discuss a few factors that convince people and businesses to adopt and use generative AI models.
Fast data processing. Generative AI systems are capable of processing huge datasets within a short time and quickly generating requested results. For instance, CarMax used Azure OpenAI Service to process thousands of customer reviews and write short summaries for each vehicle model they sell. To write so many pieces of content manually, the company would have needed years and tens or even hundreds of content writers.
Unlimited creativity. The output the generative AI model produces, of course, depends on the initial inquiry a person makes. But the model can shape this output in limitless ways and never gets tired or suffers from a lack of ideas.
High adaptability. The fact that generative AI models are capable of processing practically any input data regardless of the industry, specialization, or expertise required to understand the subject matter makes them extremely useful across departments, helping enterprises improve their services at all levels.
In the next section, we consider a few benefits you can count on when training a generative AI model with your proprietary data and when simply using pre-trained models and solutions as assistants in your daily workflow.
Explore how we developed a real-time election data processing solution.
See case studyBenefits of generative AI technology: Expanding business capabilities and potential
Section contents:
- Advancing the business workflow with generative AI
- How generative AI drives innovation and reduces operational expenses
- Ways to speed up research and development initiatives with AI models
- Challenges and risks to consider before choosing generative AI software
A generative AI model can allow your organization to evolve in multiple ways. Below, we discuss some of them.
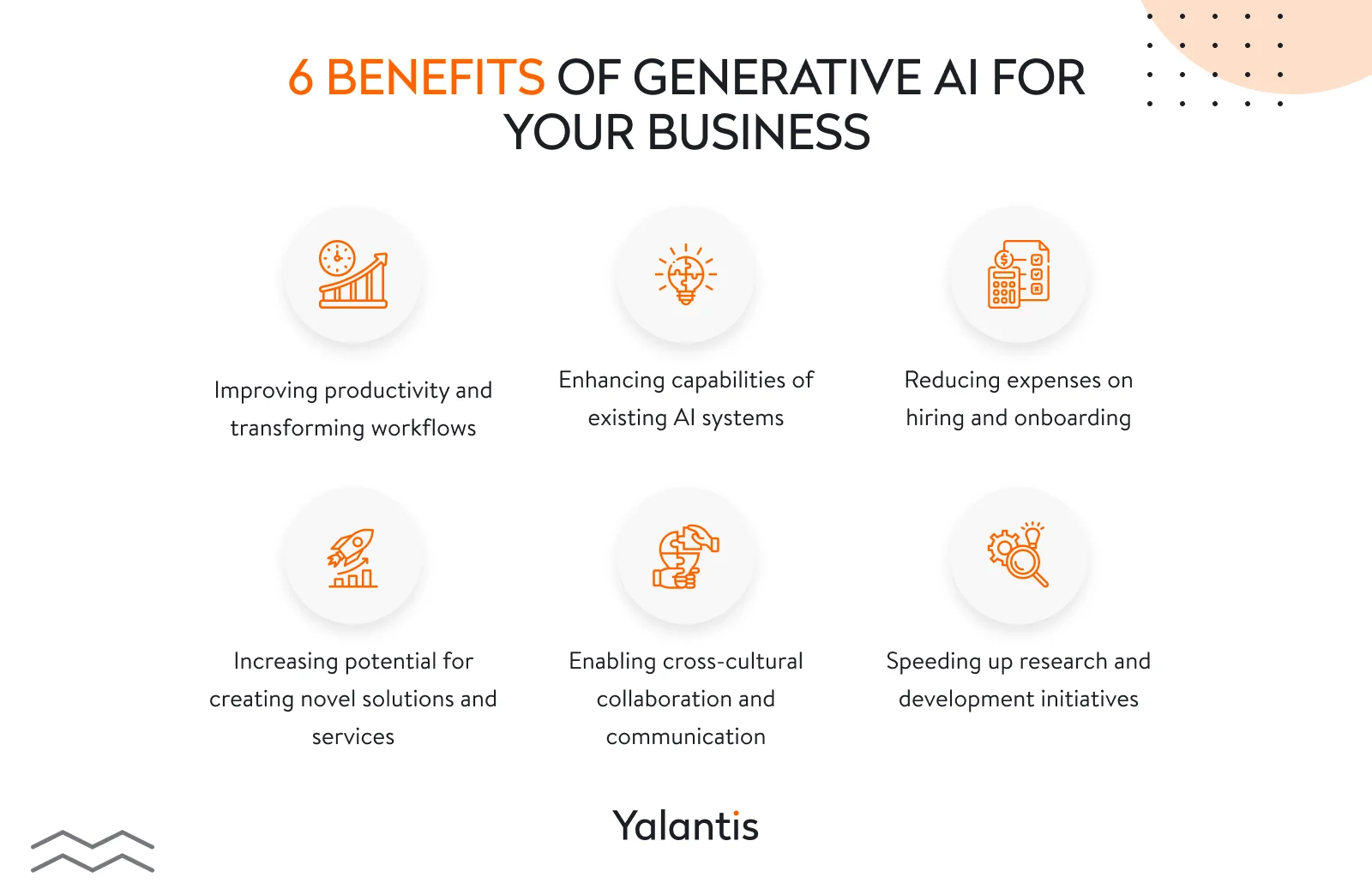
Improving productivity and transforming workflows. Generative AI models can help organizations in diverse industries streamline and optimize various processes. These models can automate repetitive and mundane tasks, generate content, analyze datasets, compose reports, assist in problem-solving, and offer insights, leading to increased employee productivity and improved business efficiency. Generative AI systems can allow employees to focus on more creative and strategic aspects of their work as well as complete their work much faster than usual.
Reducing expenses on hiring and onboarding employees. A suitable and properly trained foundation model can supplement missing expertise at your company. For example, well-trained generative AI chatbots and assistants can provide your customers with round-the-clock consulting and support services without much involvement on your side.
Enhancing the capabilities of existing AI systems. AI models can generate synthetic data that you can use for training your existing AI systems, thus improving their performance without the risk of losing sensitive business or customer data. For instance, the UK Financial Conduct Authority (FCA) has created synthetic payment data to train an AI model without risking revealing or misusing real-world customer payment information. With synthetic data, the FCA can use the full potential of generative AI to come up with an accurate fraud detection mechanism.
Increasing the potential for creating novel solutions and services. Among the many benefits of generative AI technology is the capability to uncover new opportunities for creativity, constantly come up with new ideas, foster brainstorming sessions, and improve your strategic planning. It can also be used to create marketing content and product or service descriptions, generate design prototypes, or even develop solutions to complex business problems. Generative AI’s ability to provide novel ideas can open up new revenue streams for your company and foster business development.
Enabling cross-cultural collaboration and communication. With superb possibilities in natural language understanding, generation, and translation, generative AI development company can help your company advance and improve cross-cultural communication and cooperation. It’s particularly beneficial for large enterprises with distributed multilingual teams.
Speeding up research and development initiatives. Each department in an organization often needs to perform all sorts of research to dig into market trends and changing customer needs, analyze competitors, and then develop a comprehensive report describing the situation and offering steps to take for improving current business operations. Generative AI can help with all of the above, from research to reporting.
Adopting generative AI is a rewarding endeavor that can help your organization not only improve business and employee productivity but also stay at the edge of top-notch technology and constantly generate new solutions that can help you stay afloat. Efficient decision-making is another challenge of owning a business. Having all-around assistance while making business decisions is priceless, and a generative AI model can be a true helping hand.
Challenges and risks of generative AI adoption
Despite all the generative AI benefits, adopting a generative AI model requires a responsible attitude, as there are a variety of bottlenecks associated with its implementation. Here are some of the most common:
Biased output. If trained with low-quality or insufficient datasets, the results the model produces might contain errors or bias. Thus, it’s critical to conduct regular data quality checks and ensure that you use only accurate and relevant data for training AI models.
Data privacy and security concerns. AI models may deal with highly sensitive data, and if proper security measures weren’t set up beforehand, businesses might have to deal with data breaches, data corruption, and reputational damage. This happened with Samsung when their employees leaked sensitive company information such as code snippets and meeting notes to ChatGPT. Now, Samsung is working on their own in-house AI model to be able to use all the perks of generative AI but with fewer risks.
Potential compliance issues. Since most AI applications and solutions are evolving quickly and new ones often appear, legislative bodies around the world don’t have enough time to come up with laws and regulations to safeguard businesses from potential harm. As a result, many companies decide to stay on the safe side. For instance, in Europe, banks aren’t willing to make AI services openly available for end users since they’re wary of security issues that could lead to losing money and regular clients. That’s why such entities as the European System of Financial Supervision (ESFS, a combination of authoritative institutions responsible for ensuring financial services stability and protecting customers) actively address challenges associated with AI adoption to ensure AI is safe for financial companies.
Environmental ramifications. Training of generative models requires high computational power and energy resources, which may increase carbon emissions. Constantly training new models can negatively impact the environment. Consider using custom-trained generative AI models only if you have a clear need, and consider integrating pre-trained models that AI software providers offer (such as Mostly AI, GPT-4, Jasper, Twain, Diagram, and Aqemia) whenever possible to avoid causing additional environmental damage.
Reliability and trustworthiness. Generative AI models can provide diverse results even with the same input, and it can be difficult to evaluate all the results and define which model would be most appropriate for your needs. A probable solution might be regularly updating the system or using premium versions of ready-made AI solutions to ensure that you use a model at its full capacity and that the results are as accurate as possible.
By paying due attention to all of these challenges, you can find an optimal and responsible way of adopting generative AI with the most possible benefit for your business and the least possible disruption to your company’s workflow.
Use cases for generative AI by industry that prove this technology is worth the investment
Section contents:
- Fast proliferation of generative AI solutions across industries
- What multimodal generative AI applications will be capable of
- Tasks that generative AI models can perform instead of or in collaboration with humans
The public release in 2022 by OpenAI of the ChatGPT and DALL-E 2 generative AI solutions that comprehend natural language prompts and excel in text and image generation worked as a charm to increase adoption rates of generative AI technology across industries. Practically half of all respondents (1,684 executives across industries) to a recent McKinsey survey on the use of generative AI mentioned that they had tried this technology at least once. Even though generative AI technology had been steadily developing for years before the launch of these platforms, it’s the ease of engaging with OpenAI’s models and the speed with which they generate relatively useful and accurate results that fascinate people most.
Current foundation models are evolving to become extremely multimodal so that in the future they can perceive not only written natural language prompts but also, for instance, use data from IoT sensors installed on a production line to create reports or manuals for machinery maintenance and repair. The prospects of growth in use cases for generative AI are vast, and we can expect to witness more and more fascinating discoveries in the near future.
At the moment, generative AI technology can be used for:
- text, image, video, audio, or code generation
- translating snippets of text to different languages
- improving the quality or changing the style of images, outdated documents, and photos
- summarizing information from reports or articles
- supporting customers in real time via chatbots and virtual assistants
Of course, this list isn’t exhaustive, and there are many more ways to employ generative AI systems. Let’s explore the different possibilities of generative AI technology by examining its use cases in healthcare, FinTech, and manufacturing.
Examples of generative AI implementation in the healthcare industry
Here are several ways in which generative AI can help the healthcare industry grow and improve the quality of healthcare service delivery.
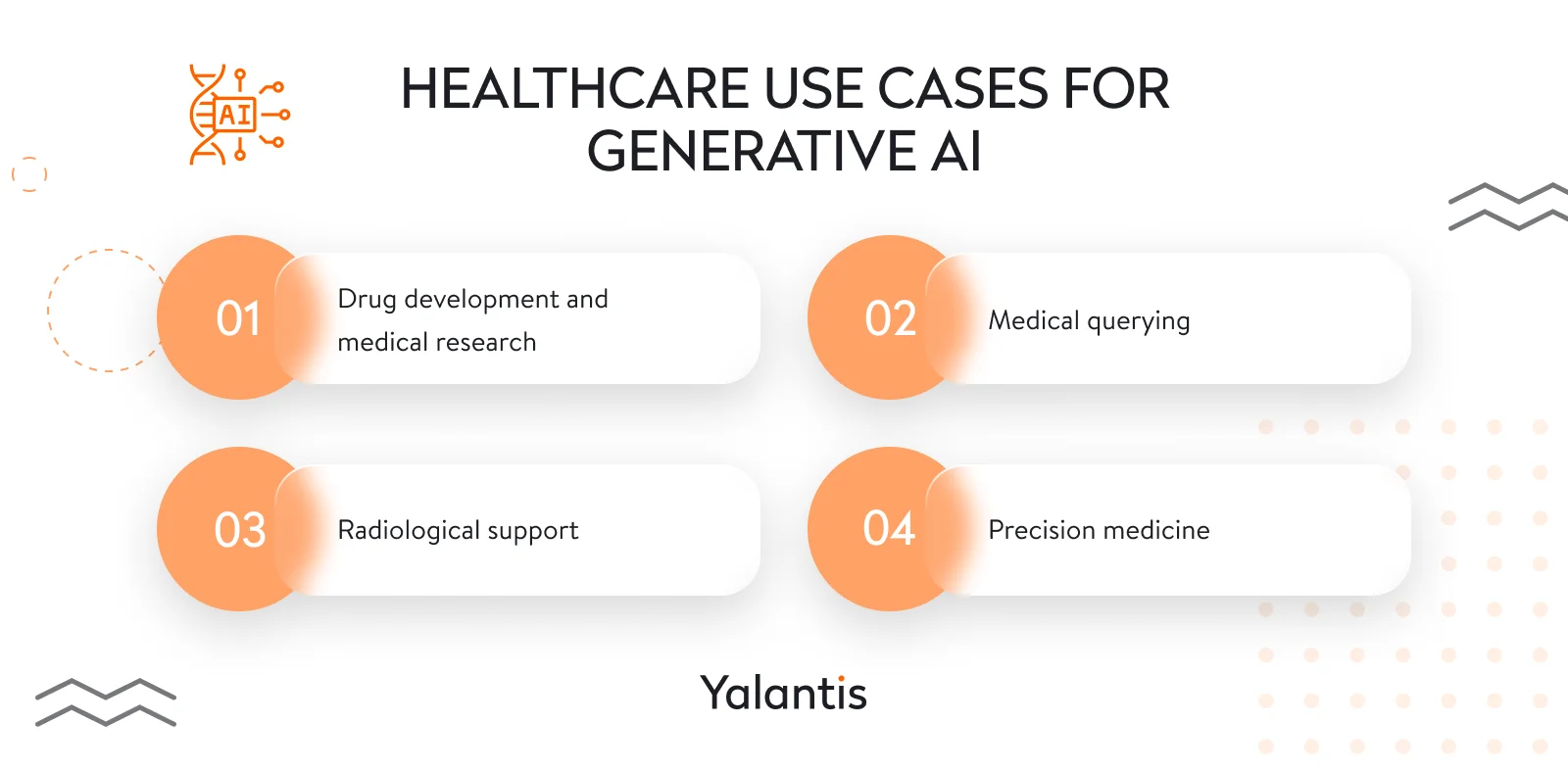
Drug development and medical research. Generative AI algorithms help pharmaceutical companies discover and test new drugs much faster than any other technology can. These models assist in protein engineering, understanding DNA sequences, and researching generics to allow medical researchers to track and define the most subtle correlations between drug components and human health. For instance, biotech startup Evozyne is using generative AI models to simulate protein evolution to discover the most suitable candidates for drug development.
Medical querying. Just like technical customer support, any health application can use the power of AI to help people and physicians get expert medical help via a chatbot. Google’s Med-PaLM is a great example of a generative AI model that provides descriptive answers to any kind of medical query and writes comprehensive summaries after processing a large amount of medical documentation. It’s a large language model (LLM) trained on medical data and evaluated by medical exams and research. Mayo Clinic physicians are already testing Med-PaLM and say that it is promising but, for now, can be used only as an assistant in the daily healthcare workflow.
Radiological support. Interpreting and analyzing medical images can be difficult, especially if their quality and resolution aren’t that high. Generative AI models can improve the quality of medical images so radiologists can make more accurate diagnoses. Plus, generative AI models can analyze medical images and write radiological reports, saving time and allowing radiologists to perform more examinations in a day.
Precision medicine. Generative AI algorithms can process large sets of patients’ medical records, prescriptions, medical images, laboratory results, and even clinicians’ notes, along with any unstructured data (lifestyle choices, handwritten health materials) to help clinicians quickly come up with personalized patient treatment plans.
If used responsibly and with caution, generative AI models can be a tremendous help in clinicians’ day-to-day workflows.
Generative AI examples in FinTech businesses
Financial businesses have vast possibilities to benefit from implementing generative models. Although AI is already actively used across this domain, even spurring the development of new FinTech applications and solutions, generative AI models allow financial companies to get a completely new perspective on running their business.
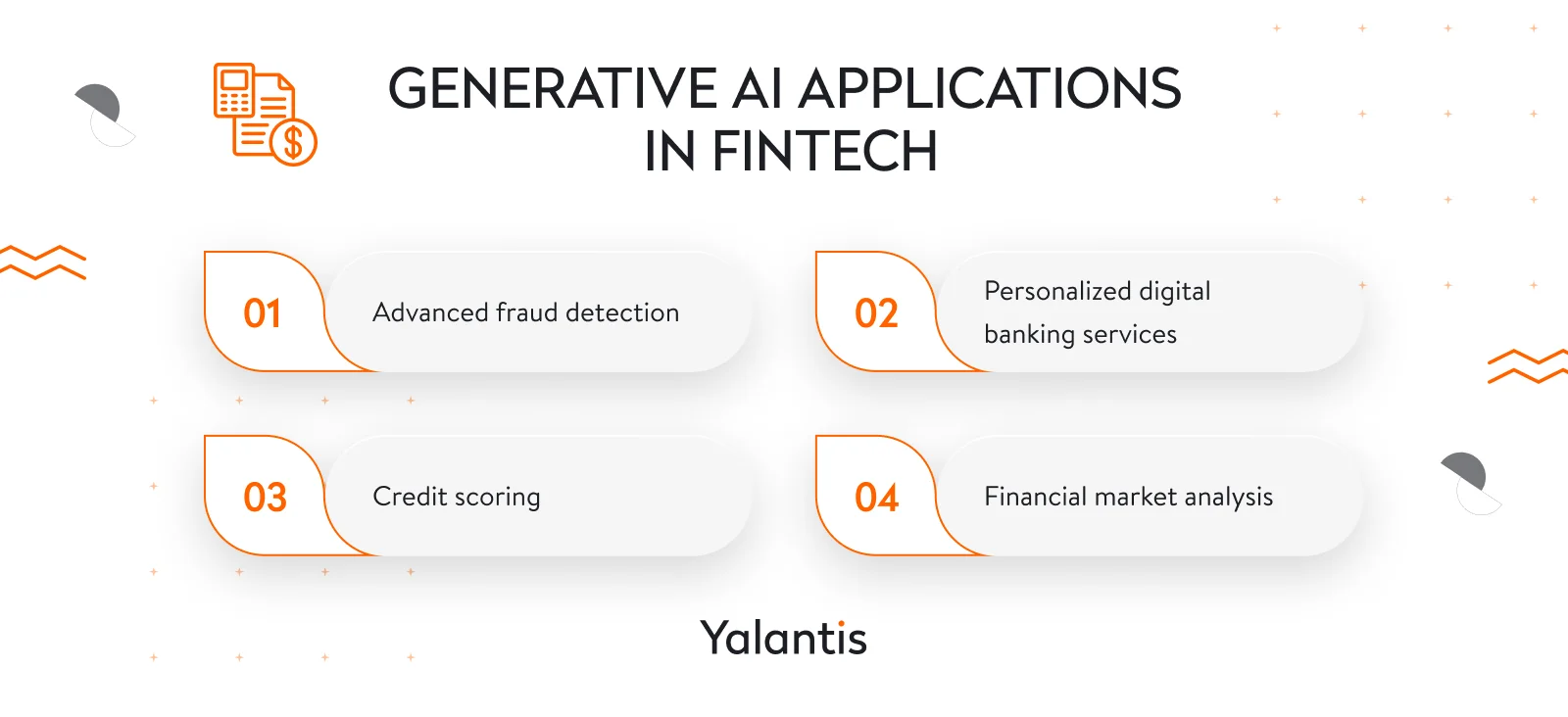
Advanced fraud detection. By analyzing large numbers of financial transactions, generative AI algorithms can not only define which transactions are potentially fraudulent but can specify precautionary measures to avoid the same situations in the future. Thus, one of the prominent benefits of generative AI models lies in the possibility of providing more extensive and detailed outputs than expected. Plus, generative AI solutions can create synthetic data for training fraud detection models and increasing their accuracy.
Personalized digital banking services. Generative AI models can help banking institutions provide consumers with highly personalized services and increase their loyalty by analyzing large datasets of structured and unstructured financial data and tracking consumer banking behavior, such as regular purchases or the frequency of international transactions. For instance, Bud Financial has launched the Bud.ai platform to provide generative AI models for hyper-personalized banking services and help financial institutions generate lots of actionable financial insights about their users.
Credit scoring. Another way of implementing generative AI is to build extensive reports about customers to verify their ability to receive loans as well as determine credit card or loan limits. For example, the Crediture platform large language models (LLMs) to process all-around structured and unstructured customer data and enable credit risk assessment even for people with practically no credit history.
Financial market analysis. Generative AI algorithms can also analyze the financial markets to predict possible trends and build economic scenarios, helping financial institutions better prepare for market changes and update their services accordingly.
Whether combined with other advanced technologies (such as advanced data analytics solutions) or used as standalone solutions, generative AI models have huge potential in the financial realm by improving and speeding up interactions between financial institutions and consumers.
Generative AI use cases for the manufacturing domain
Generative AI also has a lot to offer manufacturers, particularly in solving such time-intensive issues as unexpected production equipment outages, allocating resources for manufacturing new products, and onboarding and training production floor staff.
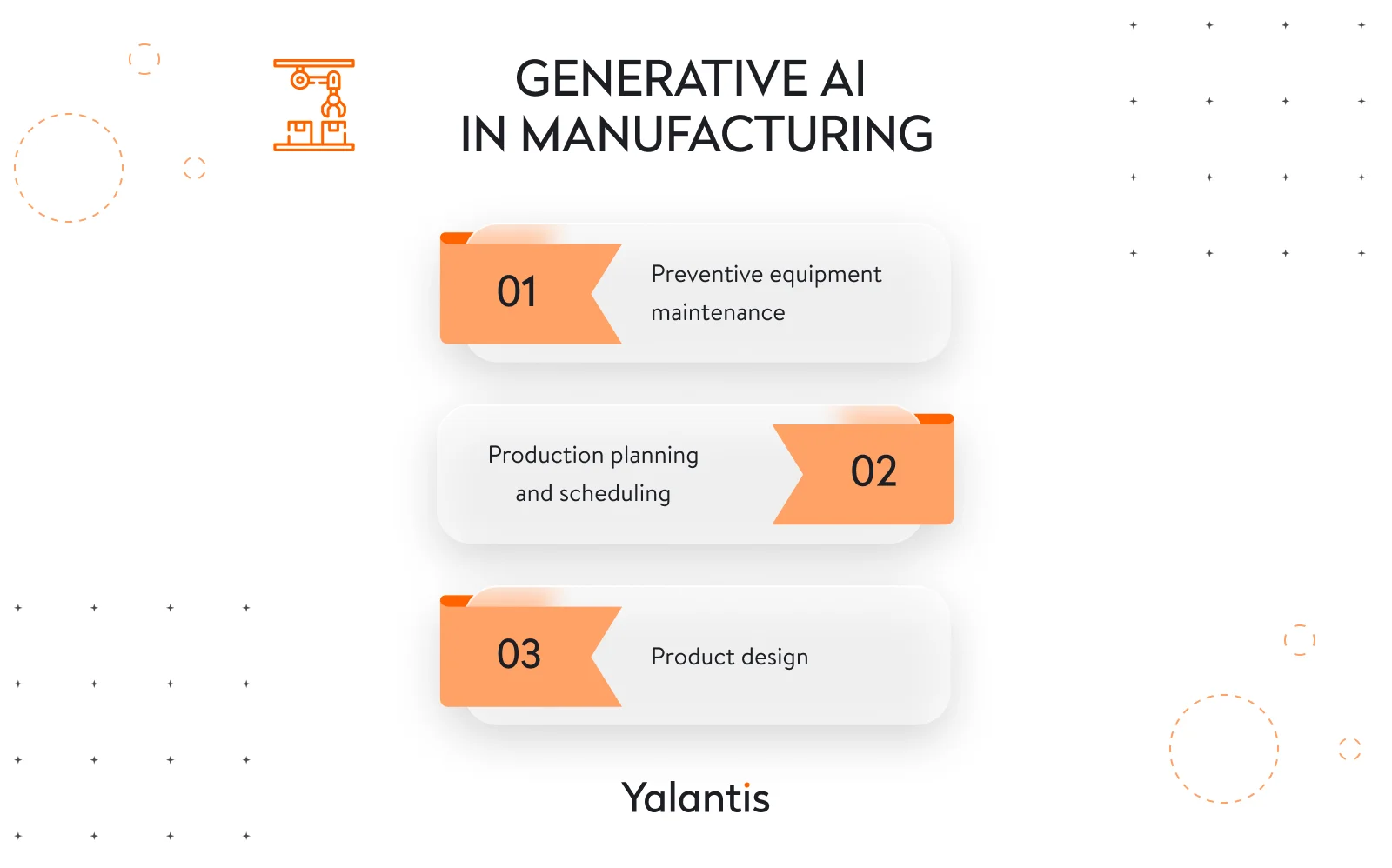
Preventive equipment maintenance. Generative AI models are capable of processing and analyzing huge amounts of machine data to quickly detect anomalies or predict equipment malfunctions as well as provide manufacturers with instructions to promptly prevent them. Plus, with generative AI algorithms, it’s possible to generate synthetic data that could include all possible scenarios of equipment failure and extensively train predictive AI models to detect even novel issues. That’s what pharmaceutical company Merck is already doing. Merck has been using synthetic defect images to train defect detection AI models with as much data as possible in order to increase the chances of detecting diverse manufacturing issues.
Production planning and scheduling. Building manufacturing reports, production plans, and schedules is often tedious and time-consuming. Generative models can automatically create reports and plans based on proprietary data and take into account lots of factors that humans may miss. BMW Group has managed to minimize idle times during assembly and efficiently meet their monthly goals using generative AI for production scheduling.
Product design. Generative models can also be helpful in creating new product designs to diversify production lines and help manufacturing companies remain competitive and in demand.
As we’ve already analyzed not only the common benefits of generative AI but also some specific benefits in the context of three separate industries, you may see that regardless of your industry, there is a way for you to reap the benefits of generative AI models. It’s just about time to start considering how to use this technology.
Things to consider before implementing a generative AI model
Drawing on years of expertise in delivering AI solutions, we’ve realized that to ensure seamless generative AI model implementation, you need to form a clear purpose for implementing generative AI, taking into account your particular business case. If it’s difficult for you to determine this purpose, Yalantis experts can perform a thorough feasibility study analyzing the pros and cons of different models to see how to most efficiently solve your business problems or meet your goals. Below are several critical aspects you should consider before implementing a generative AI solution.
- Accurate budget planning. As with any business initiative, assessing the potential return on investment is crucial. The costs associated with developing and deploying generative AI models can be substantial. Thus, you should calculate the expected benefits, such as increased productivity, revenue, or customer satisfaction, and weigh them against the upfront and ongoing costs.
- Data availability and quality. Generative AI models, especially those used in tasks like image or text generation, heavily rely on large, high-quality datasets. Therefore, it’s critical to set up a robust data infrastructure and architecture to prepare for implementing a generative AI model and ensure a smooth data aggregation process to leverage all relevant datasets.
- Social and ethical considerations. You should also take into account the ethical implications of using your business or customer data in generative AI models to avoid financial losses or reputational damage. For this purpose, you can implement staff training procedures and regularly update your employees on the rules of using generative models.
- Focus on AI model security. AI algorithms are vulnerable to adversarial attacks, which involve manipulating inputs to produce unintended and potentially harmful outputs. As part of the AI model implementation process, we focus on establishing security measures to safeguard your system against such attacks or any other malicious attacks affecting your data.
Once you have considered your goal for generative AI adoption, defined tangible benefits you can expect from it, and established a scalable and secure data infrastructure, you can proceed to selecting and implementing a generative AI model. Yalantis’ end-to-end data science services include everything you might need for smooth generative AI model implementation.
Future prospects awaiting generative AI
More generative AI applications will appear in the near future, and their accessibility will increase as AI models become available via APIs or as embedded systems. Thus, businesses will have an opportunity to customize and embed pre-trained models into their current digital ecosystems to save time and costs on training custom models.
The ever-increasing number and complexity of diverse generative AI models will require their constant fine-tuning, which in turn will require more specialists in this field. Thus, we will soon witness more generative AI experts, making it easier to hire them or consult with them. Yalantis AI engineers, in fact, are also gaining proficiency in generative AI models. If you already have an AI project in mind, reach out to us, as we’re eager to help you push the envelope and broaden your business capabilities.
FAQ
What makes generative AI so popular?
After the public release of many generative AI platforms in 2022, interest in this technology has been at an all-time high. It’s the capability of these platforms to quickly generate original content that people admire the most. Trained on large datasets created by humans, generative AI models provide brand-new results and help users solve complex issues. They can also simply work as a more descriptive and advanced Google-like search system.
How accessible is generative AI technology for businesses?
Generative AI technology is becoming more accessible to businesses worldwide due to open-source tools, cloud services, and pre-trained models. However, the degree of accessibility still varies widely, and businesses need to carefully assess their specific needs and resources when considering the adoption of generative AI technology. It’s also important to stay informed about the latest developments and best practices in the field.
Are there any notable limitations or drawbacks of generative AI in its current state?
Current generative AI models may sometimes lack understanding of inputs because the data they were trained on wasn’t sufficiently up to date. They also tend to overgeneralize and have limited control over their outputs. Additionally, their complex nature can make it difficult for humans to interpret the results, making generative AI models particularly challenging for critical applications, such as in the healthcare or legal domains. Generative AI’s computational demands, legal and ethical concerns, environmental footprint, security risks, and scalability complexities also pose challenges.
Increase business performance and customer satisfaction with generative AI.
Embark on your journey with Yalantis AI engineers
Rate this article
5/5.0
based on 41 reviews