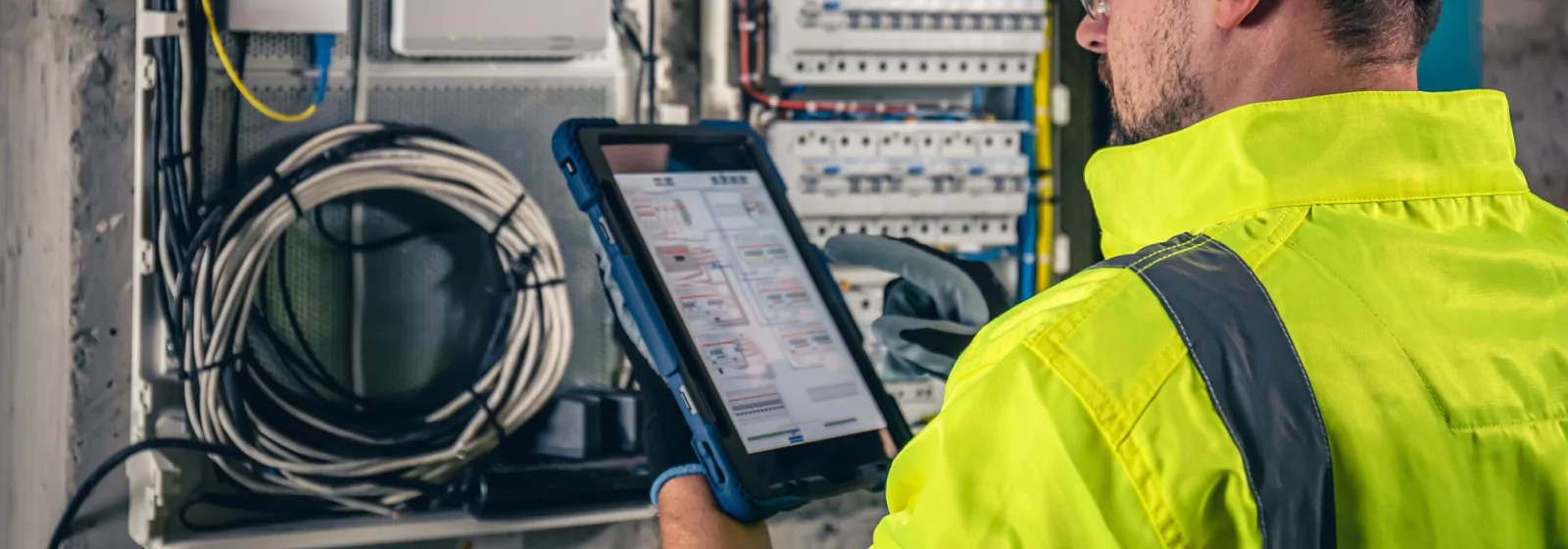
Being informed in advance: IoT predictive maintenance
Summarize:
Introduction: the power and responsibility of predictive maintenance in modern industries
With the development of technology that helps to prevent failures, still having big amounts of business failures sounds more and more unprofessional and harmful. We’re lucky to operate in the technology age, where mistakes are not that crucial – the chance of us seeing the second sinking of Titanic is very low. But what exactly can industries do to prevent unnecessary failures and avoidable spending? One of the greatest means we have now is predictive maintenance tools.
Predictive maintenance, powered by IoT, AI, and machine learning, has grounded itself as a sufficient solution to address predictable issues. By leveraging real-time and historical data, predictive maintenance helps organizations to foretell equipment failures, optimize supervision schedules, and avoid costly reduced downtime.
In this article, we will cover the fundamentals of predictive maintenance, the role of sensors, historical data, and AI/ML, see which industries benefit from predictive maintenance solutions, and go through the key benefits and challenges of predictive analytics.
What is the role of predictive maintenance systems in the IoT context?
Using predictive maintenance is a time-proven practical approach to forecast equipment failures before they occur, based on data-driven insights. IoT predictive maintenance helps to minimize unplanned downtime, extend equipment lifecycle, and, eventually, reduce overall predictive maintenance costs. The obvious advantage of predictive maintenance, in comparison with reactive maintenance, is preventing the failure, not dealing with it once it occurs. IoT-based predictive maintenance is all about exploiting real-time and historical data to predict issues accurately and optimize maintenance activities.
Predictive maintenance helps engineering personnel like CIOs, CTOs, and R&D Leads to improve businesses’ operational efficiency, allocate resources more correctly, and enhance safety.
IoT technology is the basis of predictive maintenance, which helps establish seamless physical asset integration with digital systems. IoT devices such as sensors, gateways, and cloud platforms collect and transmit real-time data on equipment performance. Further, the system helps to analyze this data, allowing decision-makers to track asset state and detect anomalies early remotely.
IoT helps make predictive maintenance highly scalable, for businesses of all sizes to be able to gain actionable insights from the hardware and machinery they operate. Moreover, remote monitoring capabilities reduce the need for frequent on-site inspections, saving both time and IoT-based predictive maintenance costs.
IoT sensors in action
IoT sensors are one of the most widely used hardware bases for predictive maintenance. A properly tuned sensor system provides real-time data, which is required for asset state monitoring. Sensors in IoT-based predictive maintenance systems usually track the following actions and states:
- Vibration: checking the vibrating patterns often helps to identify misalignment, wear, or imbalances in machinery;
- Temperature: noticeable temperature changes can signal overheating, friction, or electricity-related issues;
- Pressure: Uncontrolled changes in pressure levels may indicate leaks or blockages in IoT systems;
- Noise: Sound sensors detect acoustic effects that might reveal mechanical defects or operational inefficiencies;
- Humidity: Analyzing moisture levels is vital in environments where corrosion or contamination can damage systems.
These sensors continuously collect sensor data from machines, offering trustworthy insights regarding their performance. The time intervals might vary from milliseconds to days and weeks, depending on the asset’s criticality and functional requirements. High-frequency data collection is a common practice for systems where rapid failure could lead to significant equipment downtime or safety hazards.
Leveraging historical and real-time data
One of the key advantages of IoT-based predictive maintenance is using both historical and real-time data to predict potential issues. Historical data is fundamental for tuning predictive models, as it helps establish patterns and trends over time. It enables systems to differentiate normal states from anomalies that require intervention.
However, the real-time data adds valuable context to historical insights, which is great for creating a dynamic monitoring system. For example, a machine might show gradual temperature increases over weeks, but real-time spikes can highlight an immediate issue. Data engineering consulting services help businesses build robust data pipelines that efficiently process and analyze both historical and real-time data, ensuring accurate predictions and timely interventions.
Data analytics, based on combining these sensor data types, enables more accurate predictions, reducing the risk of false positives or missed warnings.
Boost operational efficiency with Predictive maintenance solutions
Our services – from consulting, architecture design to solution implementation will help you to track real-time data, analyze it, prevent failures and avoid downtimes.
Key metrics for monitoring
When implementing IoT-based predictive maintenance, we recommend keeping an eye on monitoring these general metrics:
- Mean Time Between Failures (MTBF): Indicates the average time a machine operates before requiring maintenance.
- Anomaly Scores: AI algorithms assign scores to data points that deviate from expected patterns, helping prioritize potential issues.
- Condition Indicators: Metrics such as vibration amplitude or thermal gradients are directly linked to the likelihood of failure.
- Operational Thresholds: Defined limits for each metric help identify when a parameter is approaching a critical state.
By monitoring these metrics, IoT-powered systems can provide actionable insights, enabling organizations to plan maintenance activities and allocate resources effectively. Additionally, IoT security implementation services ensure that the data collected and transmitted remains protected from cyber threats, maintaining the integrity and reliability of predictive maintenance systems.
Role of artificial intelligence and machine learning in predictive maintenance
Data-driven models for IoT-based predictive maintenance
AI and ML models are at the essence of predictive maintenance, enabling businesses to analyze equipment performance patterns and predict the probability of failures with precision. These models process immense volumes of data collection results by IoT sensors, which helps to identify patterns that signal potential issues.
We recognize two basic learning approaches:
- Supervised Learning: We use labeled historical data to train models. As an example, a model trained with data on equipment failures from the past can recognize specific conditions that indicate an impending fault.
- Unsupervised Learning: When ordered data is unavailable, we use unsupervised learning to detect anomalies by identifying divergences from normal operating behavior. Businesses typically utilize dimensionality reduction techniques to uncover hidden patterns in data.
AI/Machine Learning models evolve over time and become increasingly accurate as they process more historical and real-time data. Being able to learn continuously makes predictive maintenance systems highly adaptable to changing operational conditions and unique asset behaviors. Incorporating machine learning solutions development enables businesses to fine-tune these models further, providing even more precise predictions and enhancing operational efficiency. For companies looking to implement tailored solutions that integrate AI, ML, and IoT capabilities, our custom IoT solution development services offer comprehensive expertise and support throughout the product lifecycle.
Fault and error data analysis
AI-powered predictive maintenance has proven its efficiency in identifying equipment errors and diagnosing the root causes of the issues. Traditional maintenance methods often rely on manual inspections and reactive fixes, which can be time-consuming and vulnerable to human error. AI transforms this process by:
- Pinpointing Faults: AI identifies the exact location and nature of equipment issues, such as misalignment, excessive vibration, or overheating after careful sensor data analysis.
- Root Cause Analysis: AI uses advanced algorithms to trace the underlying cause of risky weaknesses, offering actionable insights to prevent recurrence.
- Reducing Human Factor: Automated fault detection and diagnosis minimizes the risk of oversight in maintenance scheduling, securing timely interventions when they are needed.
Using these approaches helps engineering teams to make data-backed decisions, which leads to better IoT-based predictive maintenance operations and reduced downtimes.
Integration with IoT systems
Businesses integrate AI and ML algorithms into IoT predictive maintenance platforms to be able to improve the automation and scalability of their solutions. This integration involves:
- Data Processing: IoT devices gather raw sensor data to be processed by AI/Machine Learning models in real time. Edge computing capacities secure low-latency analysis for the most essential applications.
- Automated Alerts: When an AI system detects anomalies or predicts failures, it automatically triggers warnings or IoT-based predictive maintenance requests. For instance, a temperature spike in a critical asset should usually stimulate immediate action.
- Maintenance Scheduling: AI optimizes IoT-based predictive maintenance schedules by predicting the best times for human intervention, which helps to minimize disruptions in operations.
- Continuous Feedback Loop: IoT platforms deliver continuous feedback to AI models, helping to refine their accuracy and adaptability over time.
Skillful operation between AI, ML, and IoT helps to deliver highly intelligent and autonomous predictive maintenance. The benefit for business here lies in reduced operational risks, improved asset reliability, and optimized resource allocation.
Revolutionize your manufacture or supply chain with Predictive maintenance solutions.
We offer custom platform development, device connectivity, and predictive tools to enhance asset performance and reduce operational costs.
Applying the IoT predictive maintenance tools across industries
Multiple branches of human activities can benefit from predictive maintenance while solving operational challenges. Below, we explore key applications across various sectors.
Logistics
Monitoring machine components
In logistics, you have to be confident in the correct work of the reliability of critical machine components like brake discs, engines, and assemblies. IoT-based predictive maintenance initiatives equipped with IoT sensors continuously monitor vibration, temperature, and wear, which helps to track the component’s health. Early detection of irregularities helps to avoid costly decompositions.
Legal compliance
It’s typical and correct for the logistics sector to have strict regulations to be sure in the safety of the vehicle. For example, upcoming legislation in Great Britain will mandate regular inspections of truck brakes, requiring detailed condition checks and reporting. IoT-enabled predictive maintenance systems can automate these reports, ensuring compliance with minimal turmoil.
Benefits
Enhancing Vehicle Safety: Regular monitoring reduces the risk of accidents caused by mechanical failures.
Preventing Fines: Compliance with safety regulations helps businesses to avoid fines and reputational damage.
Streamlining Fleet Management: Predictive data helps businesses optimize vehicle usage, schedule timely repairs, and extend the lifespans of their solid assets.
Industrial sector
Parameters for monitoring
Industrial units rely on IoT sensors to track critical state parameters like temperature, vibration, and noise. These metrics reveal the operational conditions of machinery and help identify potential issues before they escalate.
Preventing downtime
Unplanned equipment downtime is never desired in industrial settings as it may lead to significant financial losses. Predictive maintenance mitigates this risk by predicting malfunctions and encouraging proactive repairs. For example, monitoring excessive vibration in a conveyor belt system can prevent a complete production halt.
Smart buildings and smart metering
Energy optimization
Predictive maintenance continuously monitors HVAC systems to identify non-efficiency in energy consumption. IoT sensors track aspects like airflow, temperature, and pressure to predict maintenance conditions, optimize energy use, and eventually ensure smarter budgeting.
Infrastructure longevity
Predictive maintenance is widely used in smart buildings to monitor and increase infrastructure health. Sensors in elevators, plumbing systems, and electrical networks have already proven their efficiency in detecting wear and tear, enabling timely repairs and extending the lifespan of critical parts.
Smart metering
IoT-powered smart meters are also widely used to forecast IoT predictive maintenance requirements, preventing energy losses and ensuring uninterrupted service for households, business real estate, and many more. For instance, predictive analytics can identify when a meter’s battery is nearing the end of its lifecycle, so the user can replace it in due time.
Agriculture
Crop and soil monitoring
In agriculture, IoT sensors help to monitor various metrics and conditions like soil moisture, temperature, and weather conditions. This data is widely used to optimize crop management. IoT-based predictive maintenance models analyze this data to prevent irrigation system failures, improve yields, and make data-driven predictions of future crops in particular regions.
Optimizing irrigation
Predictive systems help farmers choose the best timing for irrigation, preserve water resources, and improve overall crop health. Sensors detect moisture levels and environmental changes to help schedule proper irrigation for weeks ahead.
Healthcare
Remote patient monitoring
IoT-enabled wearable devices track important body conditions such as heart rate, oxygen levels, and body temperature. Predictive maintenance tools analyze this data to detect potential health issues before symptoms become severe and also help medical workers to have patient data remotely.
Medical interference prediction
AI-powered IoT predictive models assist in forecasting health conditions like cardiovascular diseases or special bodily states like premature births. For example, continuous monitoring of heart rate variability can indicate early signs of heart disease, qualifying for preventative care.
Predictive maintenance in healthcare
By integrating IoT devices with predictive maintenance systems, healthcare providers can operate on real-time insights. For instance, a wearable device detecting irregularities in a patient’s vitals can trigger an immediate alert to the maintenance team, for them to be able to intervene when it’s needed and be prepared for every particular kind of possible intervention.
How Yalantis adds value with predictive maintenance: IoT accelerator
Understanding the importance of proper data processing and challenges of integrating IoT-based apps, we at Yalantis developed an IoT Accelerator – a domain- and cloud-agnostic data management platform with a set of pre-built features for complex data analysis. It helps IoT-related businesses increase the time to market, reduce development and operating costs, improve security, and provide a room for scalability.
For instance, the IoT Accelerator is a decent tool if your business requires a detailed vision on future electricity consumption. Sensor Data and Device Management dashboards greatly assist in monitoring things like energy consumption per building, real-time devices consumption (with the info on humidity temperature, pressure, etc, on display), connection status, location of each device, and predicting energy consumption via ML data.
The IoT Accelerator will also help with gathering real-time data from multiple sources, storing and transmitting this data, utilizing dashboards with data and analytics, and generating reports. Feel free to request a demo and see how it changes the game for your business!
Benefits of predictive maintenance for businesses
Predictive maintenance has been proving to be a game-changer for years due to empowering businesses with better operational efficiency, smarter budgeting, and sustainability. Here’s a little more detail about each benefit IoT predictive maintenance provides:
Cost savings
Spending less money on repairings and minimizing unexpected downtimes is a measurable benefit for any kind of business. More traditional reactive maintenance often results in expensive emergency repairs and prolonged downtimes, as well as preventative maintenance involves unnecessary servicing of assets. Predictive maintenance systems eliminate these inefficiencies by determining the exact moment an intervention is required.
- Reduction in Repair Costs: Timely detection of potential issues prevents minor problems from escalating into major failures. For example, replacing a defective bearing is far less expensive than repairing an entire motor assembly damaged due to negligence.
- Minimized Downtime: Addressing proactive maintenance needs helps businesses avoid unplanned shutdowns, which is obviously great for steady productivity and profit. For industries like manufacturing and logistics, even a few hours of reduced downtime can lead to significant resource flops.
IoT data analytics and visualization play a key role in predictive maintenance by transforming raw sensor data into actionable insights and real-time visualizations, making it easier for businesses to monitor asset performance and make timely decisions.
Regulatory compliance
The majority of industries operate under uncompromising regulatory frameworks that require businesses to hold equipment to specific standards. IoT-based predictive maintenance ensures compliance by continuously monitoring asset state and providing detailed reporting.
- Proactive Maintenance: Automated alerts and scheduled interventions help to make sure that the required equipment remains within legal operating parameters, reducing the risk of non-compliance.
- Audit Trails: Predictive maintenance systems generate comprehensive maintenance logs, simplifying the process of demonstrating compliance during inspections.
Adhering to regulations helps businesses avoid fines and other unnecessary spending and enhances the reputation for safety and reliability.
Improved asset lifecycle
IoT Predictive maintenance extends the usable lifespan of machinery and infrastructure by guaranteeing the assets to operate within optimal conditions. Real-time monitoring detects early signs of wear and tear, helping businesses to understand when exactly adjustments or repairs are required to prevent further degradations.
- Maximizing Asset Utilization: Properly maintained equipment lasts longer and delivers consistently, which helps to decrease the need for replacements.
- Deferred Capital Expenditures: Businesses can delay costly equipment updates by using their current assets longer.
Sustainability
IoT Predictive maintenance contributes to greener operations by optimizing resource usage and reducing waste.
- Energy Efficiency: Detecting the inefficiencies in equipment, such as motors consuming excessive energy due to misalignment, helps predictive maintenance systems to reduce energy consumption. As an example, take a look at how we at Yalantis helped a US-based agricultural engineering manufacturer reduce the environmental impact by 30%.
- Minimized Waste: Early intervention prevents serious equipment failures, which helps to avoid waste generated from scrapped materials or inoperable products.
- Environmental Responsibility: Businesses adopting IoT Predictive maintenance harmonize with responsible sustainability goals, improving the impact on the environment, which is great for the brand image.
IoT Predictive maintenance empowers businesses to achieve operational excellence while meeting sustainability targets, creating a win-win scenario. As a real-life example, see how Yalantis helped a hardware manufacturer from Southeast Asia increase annual revenue, get faster to the market, and acquire more enterprise-level customers a year with a custom IoT-based solution.
Conclusion: unlocking the potential of predictive maintenance
Predictive maintenance represents a transformative step forward in how industries manage their assets in an efficient, reliable, and sustainable way. Applying IoT Predictive maintenance practices helps businesses transition from reactive or preventative maintenance approaches to a proactive maintenance model that prevents failures before they happen. This shift is not just a technological upgrade – it’s a modern business standard if you strive to stay competitive.
Integrating IoT sensors into predictive maintenance systems helps you get valuable insight into the state of machinery and infrastructure you use for achieving your business goals. Real-time and historical data, processed through advanced AI/ML models has proven to be effective in predicting equipment failures. Internet of Things solutions enhance the effectiveness of these models by providing a seamless flow of data from various sensors, enabling accurate predictions. It helps to reduce unplanned downtime, extend the lifecycle of critical assets, and minimize repair costs. It also ensures compliance with government and industry regulations, protecting businesses from legal repercussions while keeping the reputation for safety and reliability high.
Despite the obvious benefits, implementing predictive maintenance is not without challenges. Businesses must address issues such as data integration, the cost of IoT infrastructure, scalability, and real-time data collection security. However, there are plenty of ways to mitigate these aspects through phased implementations, robust cybersecurity measures, and partnerships with experienced service providers.
FAQ
What are the biggest challenges in implementing predictive maintenance solutions?
Here are the three biggest challenges you’re very likely to face while implementing predictive maintenance into your business:
- Integrating IoT systems with existing infrastructure;
- Providing reliable data collection;
- Cybersecurity-related risks.
Also, implementing predictive maintenance will require a lot of trust in your personnel, so they analyze the data and interpret insights effectively. Some businesses also find scalability and implementation costs challenging, while working with predictive maintenance systems.
Can predictive maintenance be implemented with outdated equipment?
Yes, but it depends on the equipment’s compatibility with IoT sensors. While legacy machinery lacks built-in connectivity, retrofitting with external sensors can enable real-time monitoring. However, challenges include data accuracy, integration with modern predictive analytics platforms, and potential disruptions during installation.
Businesses often adopt a phased approach, starting with critical assets to test feasibility before expanding predictive maintenance across their operations.
In which areas is the application of predictive maintenance most appropriate?
Predictive maintenance is highly beneficial in industries where equipment reliability is critical. Key applications include logistics (fleet and warehouse automation), manufacturing (machinery uptime optimization), smart buildings (HVAC and energy efficiency), agriculture (irrigation and soil health), and healthcare (wearable patient monitoring). By reducing downtime and maintenance costs, predictive maintenance ensures business continuity, regulatory compliance, and optimized asset utilization across various sectors.
Revolutionize your manufacture or supply chain with Predictive maintenance solutions.
Rate this article
4.9/5.0
based on 15 reviews