Data management services
-
Dive deep into root causes of data pipeline issues to integrate fitting data management technologies and stop wasting resources on quick fixes
-
Ensure coordination between your datasets to match data across systems and enable efficient cross-system reporting
-
Get trustworthy and reliable business reports and insights with a consistent data management flow that eliminates data silos and errors
-
Avoid data risks associated with growing data volume and changing business requirements by scaling data quality processes and maintaining consistent data quality
-
Ensure secure and responsible AI adoption by implementing advanced data management practices and data technologies that facilitate cross-company AI use
Real-life results of well-established data management practices
-
Up to 50%
improvement in data quality through robust validation and standardization
-
Up to 40%
faster decision-making with unified and centralized data management solutions
-
Up to 99.9%
compliance with industry standards (e.g., GDPR, HIPAA)
-
Up to 30%
cost reduction in data management operations through efficient processes
Data management services Yalantis provides
-
Data architecture services
Data architecture services
- Choosing a data architecture framework
- Developing a high-level plan for managing data assets
- Building, maintaining, and optimizing data pipelines
-
Data modeling and design services
Data modeling and design services
- Structuring business data
- Selecting data modeling techniques and approaches
- Implementing tools for efficient data modeling
-
Metadata management services
Metadata management services
- Implementing metadata repository and data catalog
- Selecting metadata management tools and technologies
- Enabling automated data discovery
- Reviewing and updating metadata strategy
-
Data quality and master data management (MDM) services
Data quality and master data management (MDM) services
- Defining data quality metrics
- Enforcing data validation rules
- Performing regular data quality checks
- Enabling data profiling
- Organizing business documentation
- Defining master data (business-critical data entities)
- Building a single source of truth
- Ensuring consistent data handling in key business entities
-
Data compliance services
Data compliance services
- Providing data management consulting
- Researching data needs and usage
- Establishing data ownership rules
- Composing data policies
- Implementing data access controls
- Enabling data compliance with industry laws, rules, standards, and regulations
- Standardizing data dictionaries
-
Cloud data management services
Cloud data management services
- Securing data storage in the cloud
- Managing data across several cloud providers
- Establishing data access rules in the cloud
- Migrating data from the cloud to on-premises or another cloud provider
-
Post-deployment support and optimization services
Post-deployment support and optimization services
- Updating and optimizing data policies
- Upgrading data architecture, data management tools and technologies
- Rolling out security patches
- Validating data accuracy, completeness, uniqueness, and relevance
- Preparing documentation and training materials for in-house data management
Data management use cases across industries
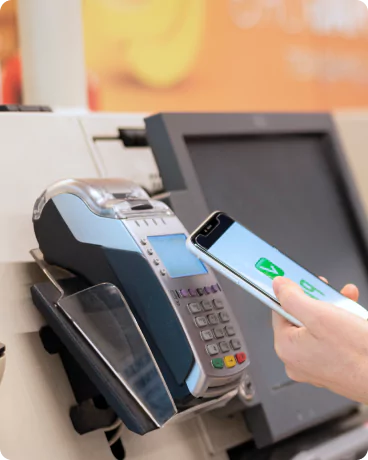
Finance
Centralizing customer and transactional data while ensuring compliance with regulations such as GDPR and PCI-DSS.
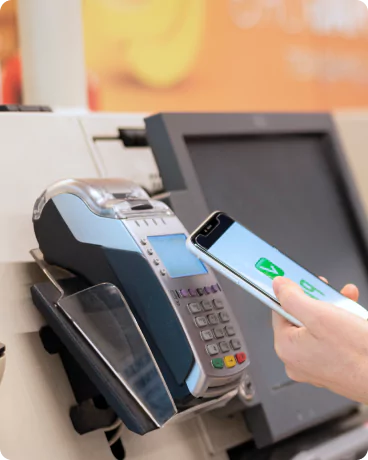
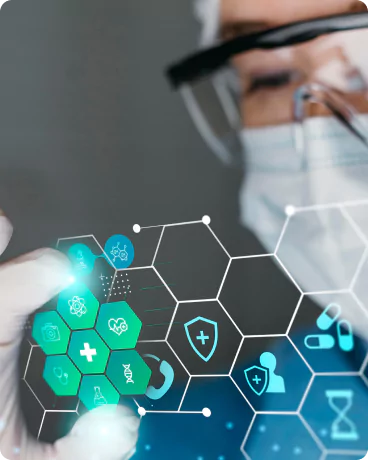
Healthcare
Streamlining patient record management across EHR systems, laboratory databases, and administrative platforms for holistic patient history overview.
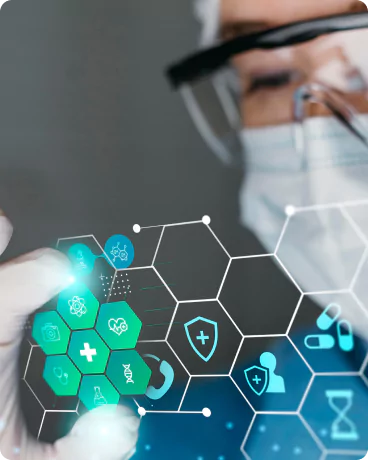
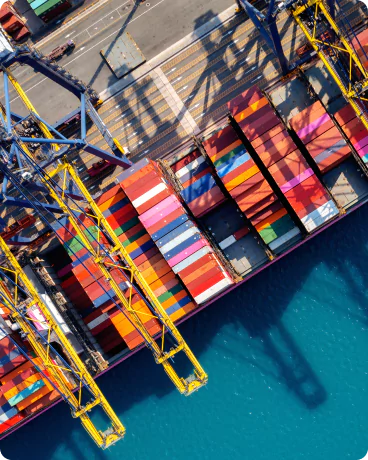
Supply chain
Organizing and managing IoT sensor data, shipment records, and fleet tracking information for improved operational insights.
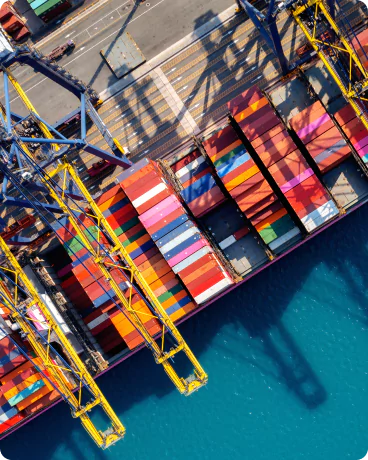
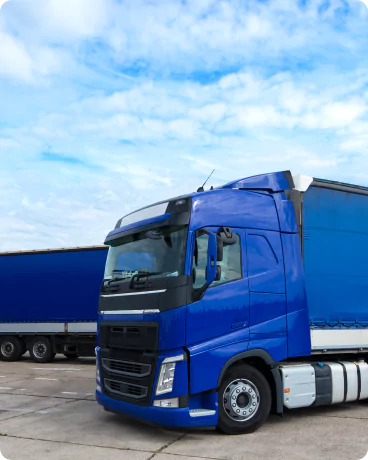
Manufacturing
Ensuring consistent quality and accessibility of production data across multiple factory locations.
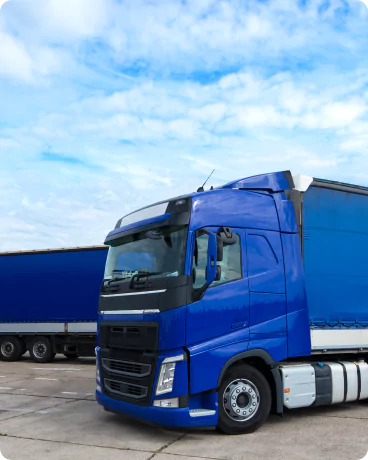
Yalantis: A company with a proven portfolio
Insights from an experienced data management service provider
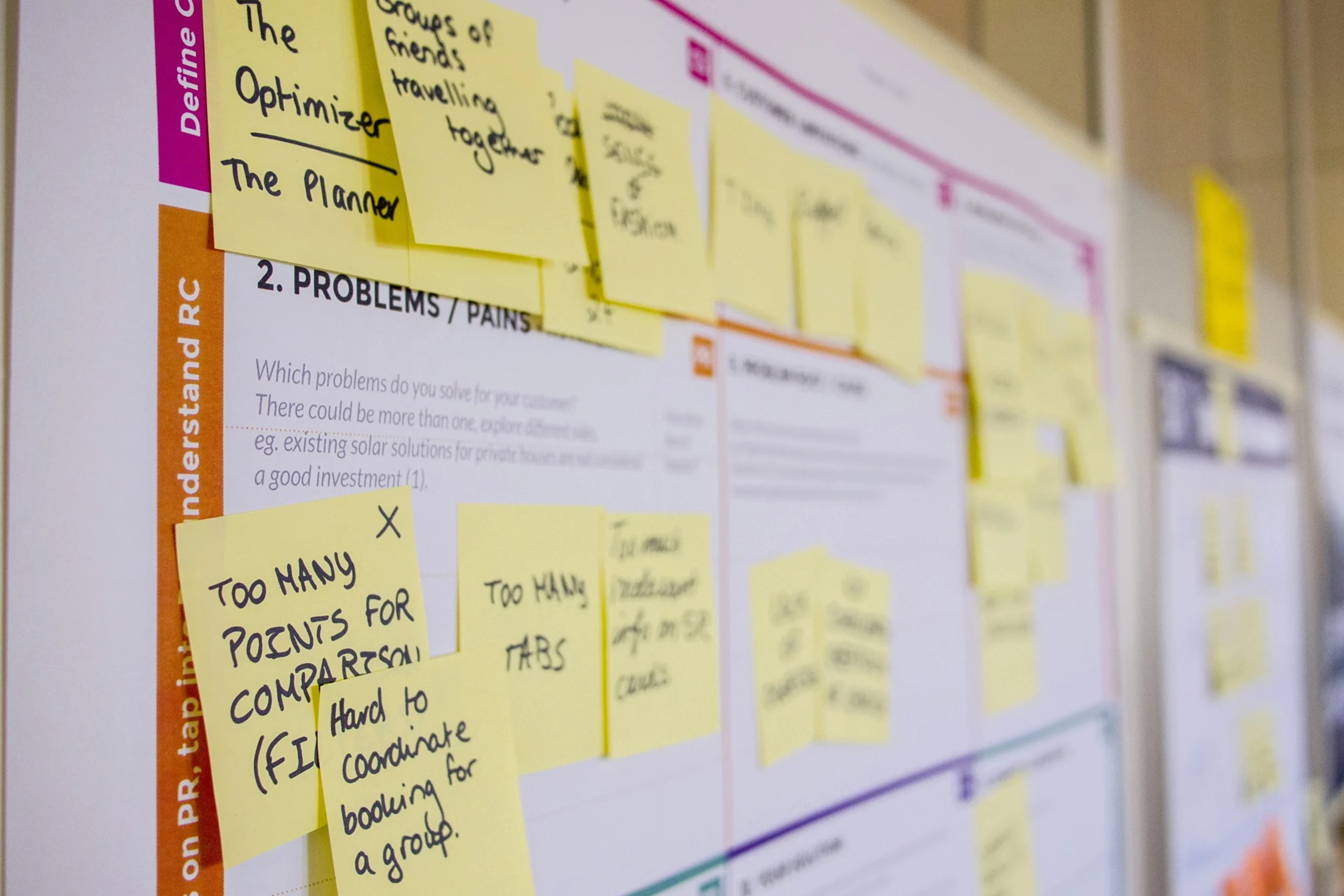
A Complete Guide to Data Modeling with Examples
Learn what data modeling is and why building accurate data models is important for software development success. Get to know why as a C-level executive you should participate in the data modeling process.
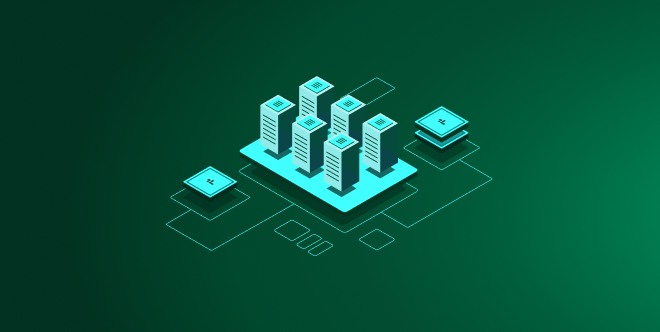
7 best practices in metadata management for IT leaders and decision makers
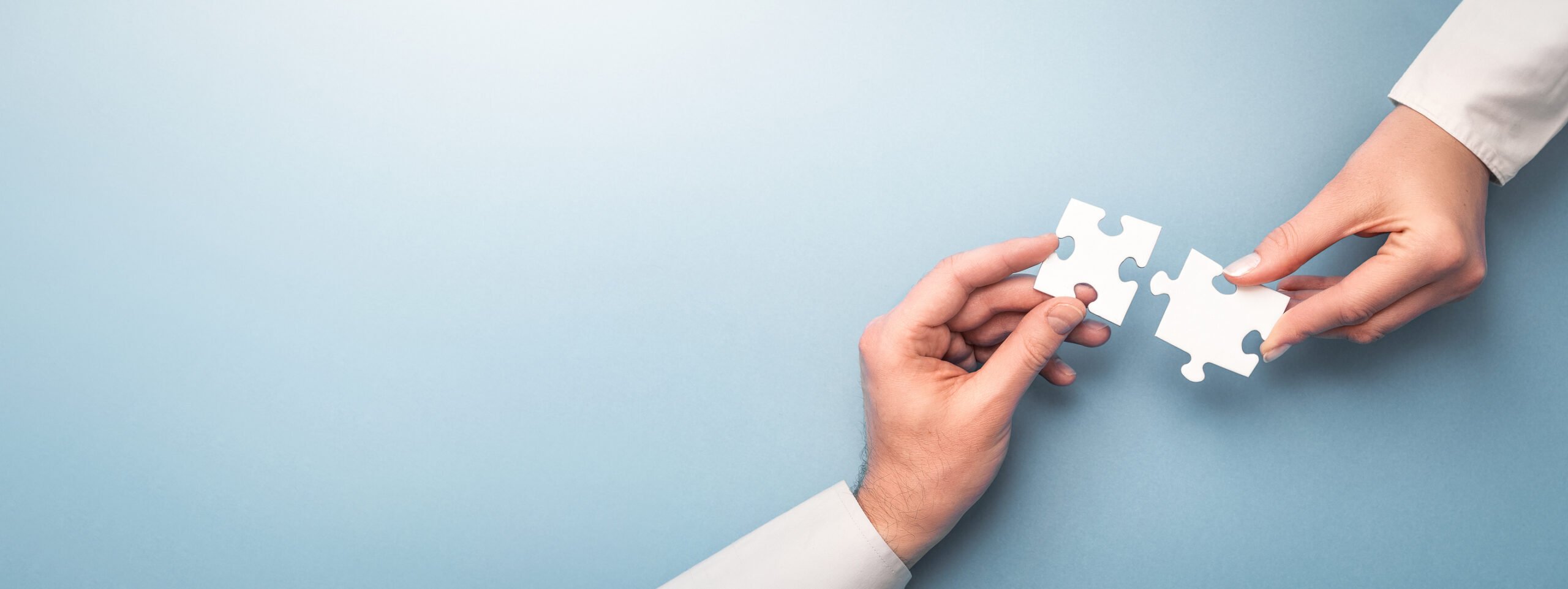
An enterprise data governance framework: Solve your biggest challenges with data security, quality, compliance
Is data chaos costing your business growth and innovation? Discover how to tackle data challenges around security, consistency, quality, and compliance with a single solution.
What is the difference between master data management and metadata management?
Master data is critical datasets on which your business services directly depend. For example, it can be your customer, product, or supplier data. The most typical systems that store such data are CRMs and ERPs. Metadata, on the contrary, is the data about your data such as column names, or date of data collection. However, master data also has metadata and one can’t function without the other.
Metadata management and master data management services are completely different and require different skills and technologies. For instance, the AWS Glue Data Catalog is a tool for building a metadata repository and catalog to overview data structures and relationships. In contrast, MDM requires various tools, particularly data integration tools, to consolidate master data from diverse sources.
How do you ensure compliance with regulations and laws like GDPR or HIPAA?
Our data security and compliance teams thoroughly study industry regulations and provide data management consulting services. For instance, the HIPAA law includes technical safeguards and the HIPAA Privacy Rule necessary for protecting electronic patient health information (ePHI). Technical safeguards contain strict access and audit controls to ensure that only authorized users can access patient data. The Privacy Rule includes security measures necessary for protecting the use and disclosure of ePHI. Strictly following every rule and recommendation listed in industry standards, laws, and regulations is the ultimate way of remaining compliant and avoiding hefty fines.
What tools and frameworks do you use for on-premises and cloud data management services?
There are numerous tools and technologies for delivering quality data management services. The choice depends on data volume, collection speed, storage capacity, and budget constraints. For cloud-based solutions, we often use tools like AWS, Azure, or Google Cloud, depending on the client’s preferences and use cases. For instance, with AWS, we can implement a managed data lake using services like Amazon S3, Glue, and Athena. These enable seamless storage, integration of structured and unstructured datasets, and support for both real-time and batch processing. Additionally, data can be automatically transferred to analytics tools like Amazon QuickSight, Tableau, or Power BI for visualization and reporting.
For on-premises environments, we design and build custom data pipelines using tools that prioritize security and compliance. Technologies such as Apache Kafka, Hadoop, or Talend are common choices for data integration and processing. Another option is hybrid data infrastructure. For example, for a healthcare client, we created a hybrid system where sensitive patient data was stored on-premises in a hospital infrastructure, while non-sensitive datasets were processed in the cloud. We ensured secure communication between the two environments using encrypted data channels, with access restricted to authorized personnel.
How do you improve data quality across multiple sources?
Data quality stands on six critical pillars: accuracy, completeness, timeliness, uniqueness, consistency, and relevance. Our data management service team selects a suitable approach for maintaining data quality that helps ensure that data is accurate, complete, timely, relevant, and unique. Such approaches can include:
- establishing cross-company data quality metrics to regularly monitor data quality
- data governance policies that set clear rules for safe data use and ownership
- keeping up-to-date business documentation
- implementing automated data validation techniques (format validation,
- range constraints, referential integrity rules)
integrating data cleansing tools
How long does it take to implement a data management solution?
The timeline for implementing a data management strategy depends on the level of the company’s data maturity. If your goal is to set up an efficient data management process on the level of a single software solution, then this can take from six months to a year. However, if you plan on revamping a data strategy across your entire organization, it can take several years and we advise you to approach this task in iterations to save resources and reduce expenses.
Role of data management in driving business efficiency
Data is a supportive force of digital transformation initiatives. For instance, if you want to implement AI—setting up seamless data management is the first step. Well-organized high-quality data is an asset that helps businesses:
- increase employee productivity as they know where to quickly find relevant data
- retain customers when customer data is timely processed and analyzed to drive personalized customer services
- scale business to new locations and regions with a transparent understanding of business processes and budget capabilities
Strategic business value of data management services
Efficient data management services involve optimizing data collection, organization, storage, processing, and analysis to ensure data accessibility, accuracy, and security throughout an entire data lifecycle. Here’s how data management drives efficiency:
- Informed decision-making. Reliable data provides businesses with the insights needed to make data-driven decisions. Whether it’s forecasting ROI, suggesting ways for optimizing the supply chain or understanding customer behavior, accurate data enables business leaders to act with confidence. Switching from data management as a service to data as a product mindset helps businesses understand that their data just like a consumer product should be managed, altered, and improved to meet user needs. Treating data as a valuable asset opens up lots of opportunities for improved business performance.
- Streamlined operations. With data management services, you can eliminate data redundancies, ensure high data quality, and optimize daily workflows. For example, manufacturing companies using integrated data management solutions can ensure automated data exchange between the manufacturing execution system (MES) and inventory management system (IMS) to help operators compose comprehensive reports and eliminate the need for manual search for correct information.
- Enhanced customer experience. Access to accurate customer data allows businesses to personalize experiences, resolve issues proactively, and predict future needs. This fosters customer loyalty and enhances brand value.
- Consistent regulatory compliance. Efficient data management ensures adherence to industry standards and legal requirements, such as GDPR, HIPAA, or PCI DSS, reducing the risk of fines and reputational damage.
- Scalability. As businesses grow, the volume of data they handle increases. Scalable data management processes prevent bottlenecks and performance downtime, allowing organizations to adapt quickly to new challenges and opportunities.
7 challenges associated with poorly devised data management processes
Some common pitfalls include:
- Data silos. When departments operate in isolation, data becomes fragmented. Siloed data makes it difficult to access holistic insights, and achieve consistent cross-company services and productivity, leading to inefficiencies and misaligned strategies.
- Technical debt. Short-term decisions to fix data issues can decrease data quality over time, leading to technical debt which weighs businesses down and prevents them from building a data-driven company. Plus, employees and leadership may lose trust in data and rely more on guesswork when making decisions.
- Lack of data governance. Without clearly defined data ownership rules across the organization, it’s difficult to monitor the use of data assets and accurately distribute responsibilities. Data governance is also about keeping continuous documentation. For instance, when data engineers who work on data pipelines leave the company without properly documenting the process, changing or managing these data pipelines can become an issue.
- Inaccurate data and over-reliance on legacy systems. Business decisions based on incorrect or incomplete data can result in costly mistakes. Such data can live and thrive in outdated systems without proper data validation protocols. And when these problematic datasets corrupt other systems, businesses get trapped in data chaos, without understanding what caused such a situation.
- Lack of skilled experts. Effective data management demands specialized expertise, but a shortage of skilled professionals—such as data engineers, analysts, and scientists—can make it challenging to turn raw data into insights. However, building an in-house team with all these roles is resource-intensive and may not be the most efficient approach. Partnering with a reliable data management service provider offers a scalable, cost-effective solution, ensuring you have the right expertise without the overhead of full-time hires.
- Cybersecurity risks. A lack of data management throughout the entire data lifecycle, including collection, storage, and sharing, makes the organization more vulnerable to cyberattacks.
- Resistance to change. Employees and leadership may resist adopting new data management solutions due to fear of complexity, costs, or perceived risks. Increasing data literacy across the organization can help your employees better grasp the importance of data management. Data management consulting experts can outline before-and-after scenarios accompanied by clearly calculated ROIs to convince business leaders that setting up data management solutions and processes isn’t a waste of resources, but a well-planned long-term plan.
Dispelling common myths about data management
Despite its importance, continuous investment in data management is often misunderstood. Many established businesses hold on to myths that prevent them from using the full potential of their data. Let’s address these misconceptions and debunk them with real-world examples:
Myth 1: “Our legacy systems are good enough.”
Reality: Legacy systems often fail to scale with business needs and lack the flexibility to integrate with modern tools. For example, Blockbuster’s refusal to adopt digital data-driven strategies contributed to its downfall. Netflix, in contrast, used data analytics to personalize recommendations and optimize content production, propelling it to industry dominance.
Myth 2: “Data management services are too expensive for mid-sized businesses.”
Reality: While implementing a cross-company data management system has upfront costs, the ROI is substantial. Consider the case of HelloFresh (a German meal-kit company), which established a centralized data management and analytics setup to optimize the supply chain, forecast customer demand, and reduce food waste and delivery costs. Increasing data maturity helped the company to operate their business at scale. In 2019, they delivered 37.5 million orders, and in only two years the number increased to 117.3 million orders in 2021.
Myth 3: “Data management is purely an IT responsibility.”
Reality: Effective data management is a company-wide effort. For example, Procter & Gamble implemented a data-driven culture by training all employees—not just IT teams—on the importance of analytics. This cross-functional approach enabled P&G to cut costs and improve product innovation.
Data management is not merely an operational function—it’s a strategic enabler of business efficiency. Whether you’re a mid-sized enterprise or a global corporation, the key to sustainable growth lies in building a data management framework that is adaptable, secure, and aligned with your organizational goals.
The cost of inaction is high. Companies that fail to modernize their data management processes risk falling behind their competitors, losing customer trust, and missing out on valuable opportunities. The good news? It’s never too late to start. Take inspiration from industry leaders, invest in the right tools and talent, cooperate with expert data management companies, and establish efficient data management to transform your business operations.
Contact us
Tame data chaos with an iterative custom data management strategy
got it!
Keep an eye on your inbox. We’ll be in touch shortlyrnMeanwhile, you can explore our hottest case studies and readrnclient feedback on Clutch.
See Yalantis reviews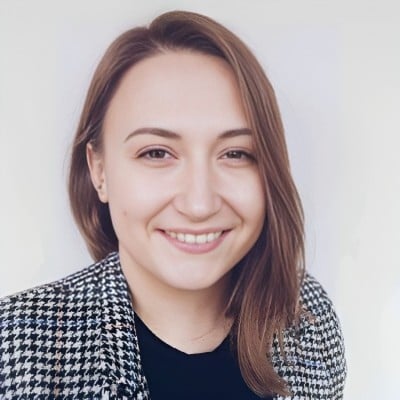
Lisa Panchenko
Senior Engagement Manager
Your steps with Yalantis
-
Schedule a call
-
We collect your requirements
-
We offer a solution
-
We succeed together!